A Queueing Model and Analysis for Autonomous Vehicles on Highways
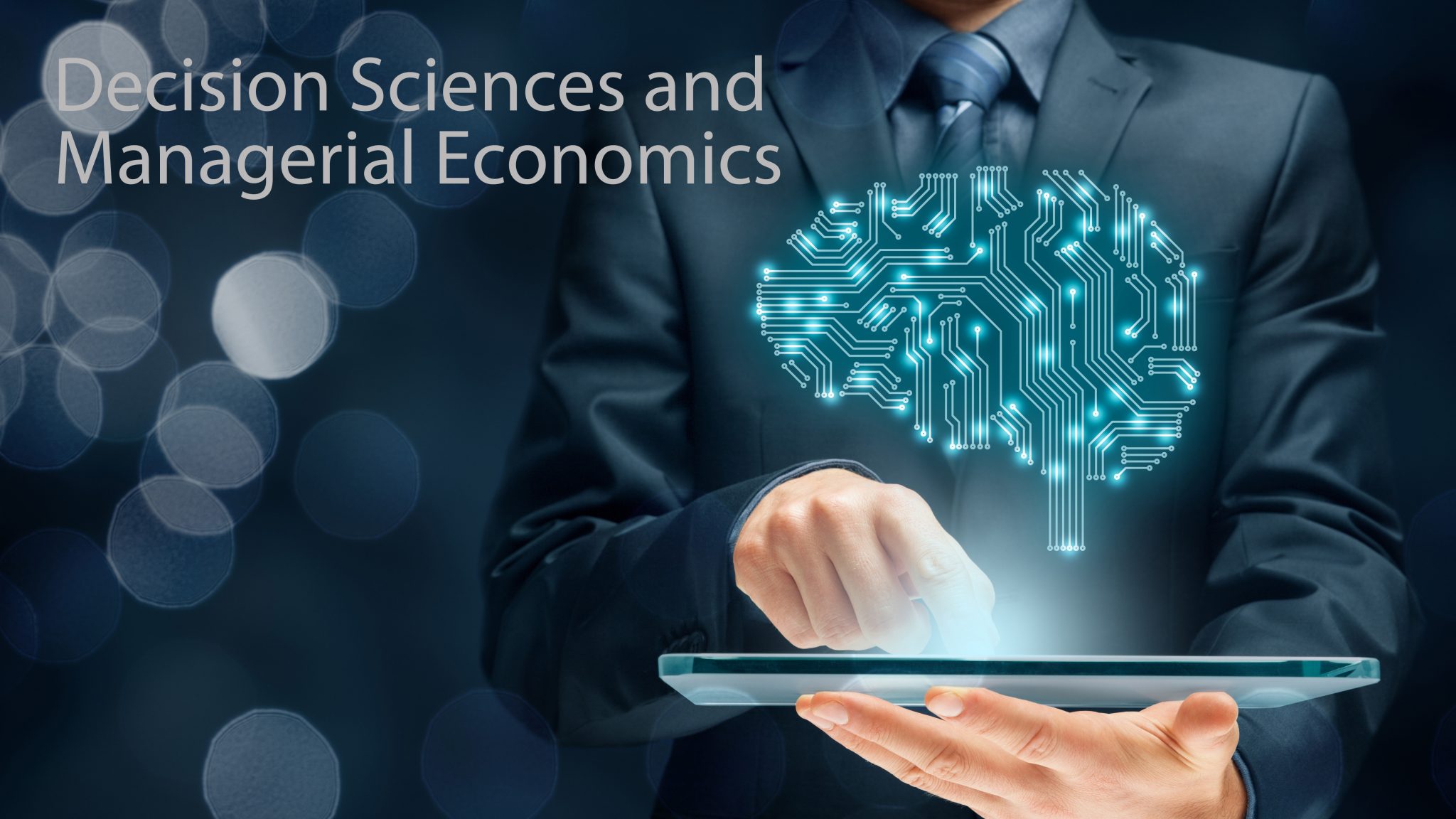
We investigate the effects of autonomous vehicles (AVs) on highway congestion. AVs have a potential to significantly improve highway congestion, since these vehicles are able to maintain smaller inter-vehicle gaps, and travel together in larger platoons (or batches) than human-driven vehicles (HVs). Various policies have been proposed to regulate AVs on highways, yet no in-depth comparison of these policies exists. To address this shortcoming, we develop a queueing model for a multi-lane highway, and analyze two policies for a mixed fleet of HVs and AVs: the designated-lane policy under which one lane is designated to AVs, and the integrated policy under which AVs travel together with HVs. In our analysis, we connect the service rate of the queueing system to congestion on the highway, as well as the inter-vehicle gaps using a Markovian arrival process (MAP). We calibrate our model to data, and we measure the performance of these policies by mean travel time and throughput. We show that, whereas the integrated policy performs at least as good as a benchmark without AVs, the designated-lane policy outperforms the benchmark only when the highway is heavily congested and AVs constitute the majority of vehicles. Moreover, this policy performs better in terms of throughput than mean travel time. Based on these findings, we recommend employing the integrated policy for a lightly loaded highway or for a highway with a significant portion of AVs; otherwise, a policy maker should carefully consider a trade-off between these two measures that depends on the AV adoption rate and various highway characteristics.