Advancements in Dynamic Matching: Approximation Schemes and Simple Policies
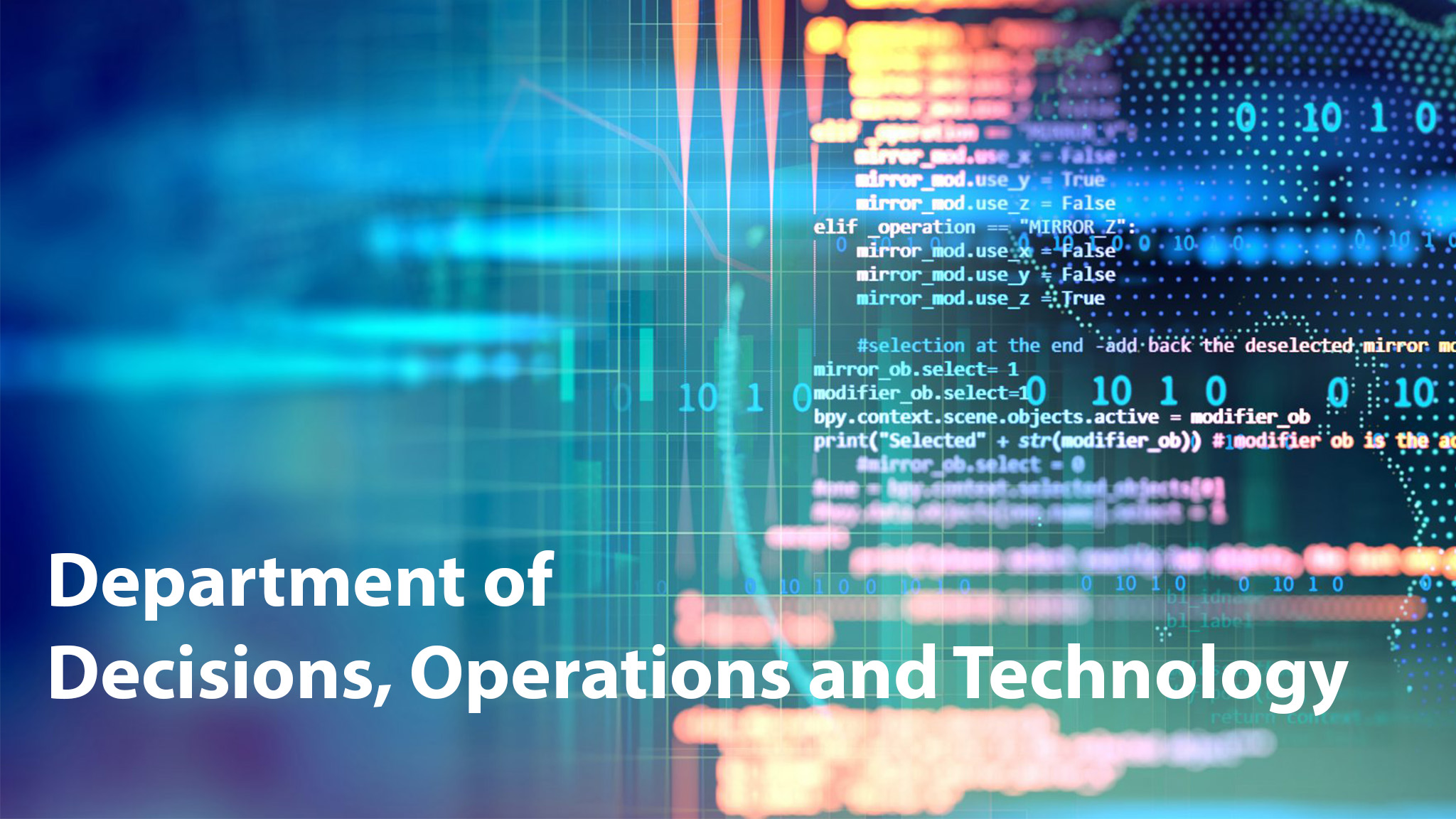
In many real-world markets and supply chains, the central planner does not control the availability of suppliers or resources. Instead, the “market thickness” is the result of a dynamic process, which affects matching efficiency. Motivated by ride-hailing, digital labour markets in the gig economy, and organ donation schemes, we study the dynamic (or stationary) bi-partite matching problem. Customers arrive according to a Poisson process and must be immediately matched to available suppliers within a network of queues. Suppliers replenish independently and abandon the market after some time.
This talk will present new results on the design and performance analysis of matching policies. Previous literature concentrates on static policies—where the matching decisions do not use real-time information. We develop a tighter linear programming (LP) framework that enables near-optimal adaptive matching policies. Our main result is a fully polynomial-time approximation scheme for both Euclidean networks and small networks. A key insight is that the network can be roughly divided into a “thin” market and a “thick” market. Our LP-rounding approach is hybrid as it combines dynamic programming for the “thin” component and fluid drift analysis for the “thick” component.
Additionally, we will briefly mention improved results for the general case using simple adaptive policies. Each supplier may randomly propose to match with an arriving customer, who selects the best proposal. Modelling the correlation structure in the design of the proposals and the analysis of the system is critical for beating the best-known performance guarantees.
Our theoretical findings pave the way for developing more efficient and effective matching policies applicable to a range of dynamic, real-world markets.