Algorithmic Pricing with Protected Consumer Characteristics
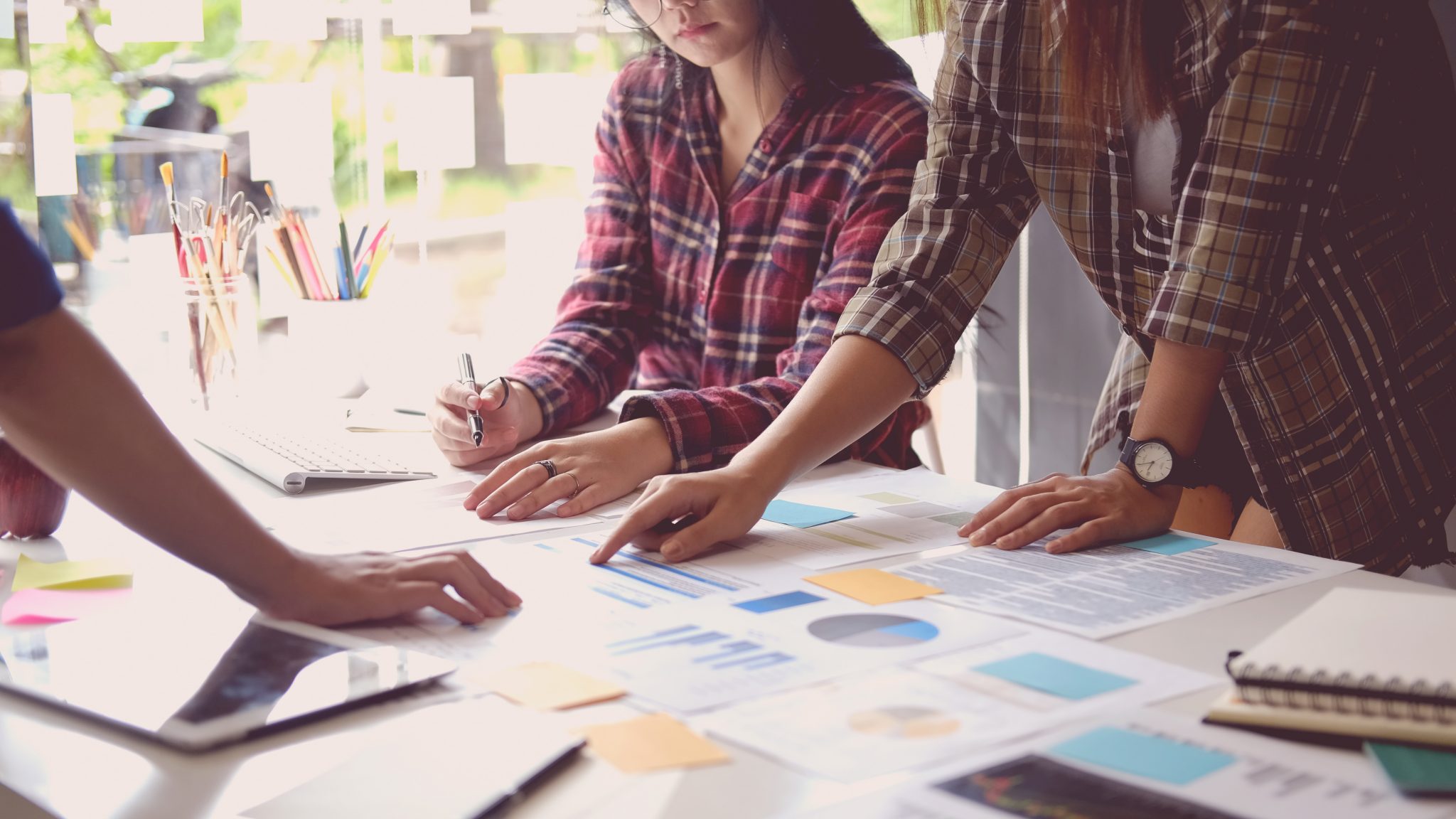
With the growing adoption of algorithmic pricing, its potential bias against certain demographic groups (e.g., minorities or women) has become a rising concern. This paper investigates two approaches aimed at ensuring fairness in algorithmic pricing and examines their implications for firm profits and consumer welfare. Specifically, the “Ban” approach prohibits the use of protected characteristics, like race or gender, in pricing algorithms. However, biases may persist, as demographic data often correlates with other factors. In contrast, the “Parity” approach allows the use of all information but requires that average prices be equal across demographic groups.
We employ a stylized model in which a firm uses algorithmic pricing to target consumer groups with different willingness to pay. The accuracy of targeting may depend on the availability of demographic data as well as other individual-level information (e.g., purchase history). Our findings suggest that Parity can lead to a Pareto improvement relative to Ban, benefiting both the firm and consumers. This occurs when demographic information complements other targeting data, especially when the protected demographic group is small (e.g., an ethnic minority) but more vulnerable to surplus extraction due to targeted pricing. Thus, Parity could be a favored regulatory approach to achieve fair algorithmic pricing, especially for minority groups at risk of price discrimination. We demonstrate that these results remain robust when the firm endogenously invests in improving targeting accuracy. Furthermore, in scenarios where consumers opt-in to personalized pricing, Parity may further enhance welfare by promoting consumer participation.