An Affine-Subspace Shrinkage Approach to Choice-Based Conjoint Estimation
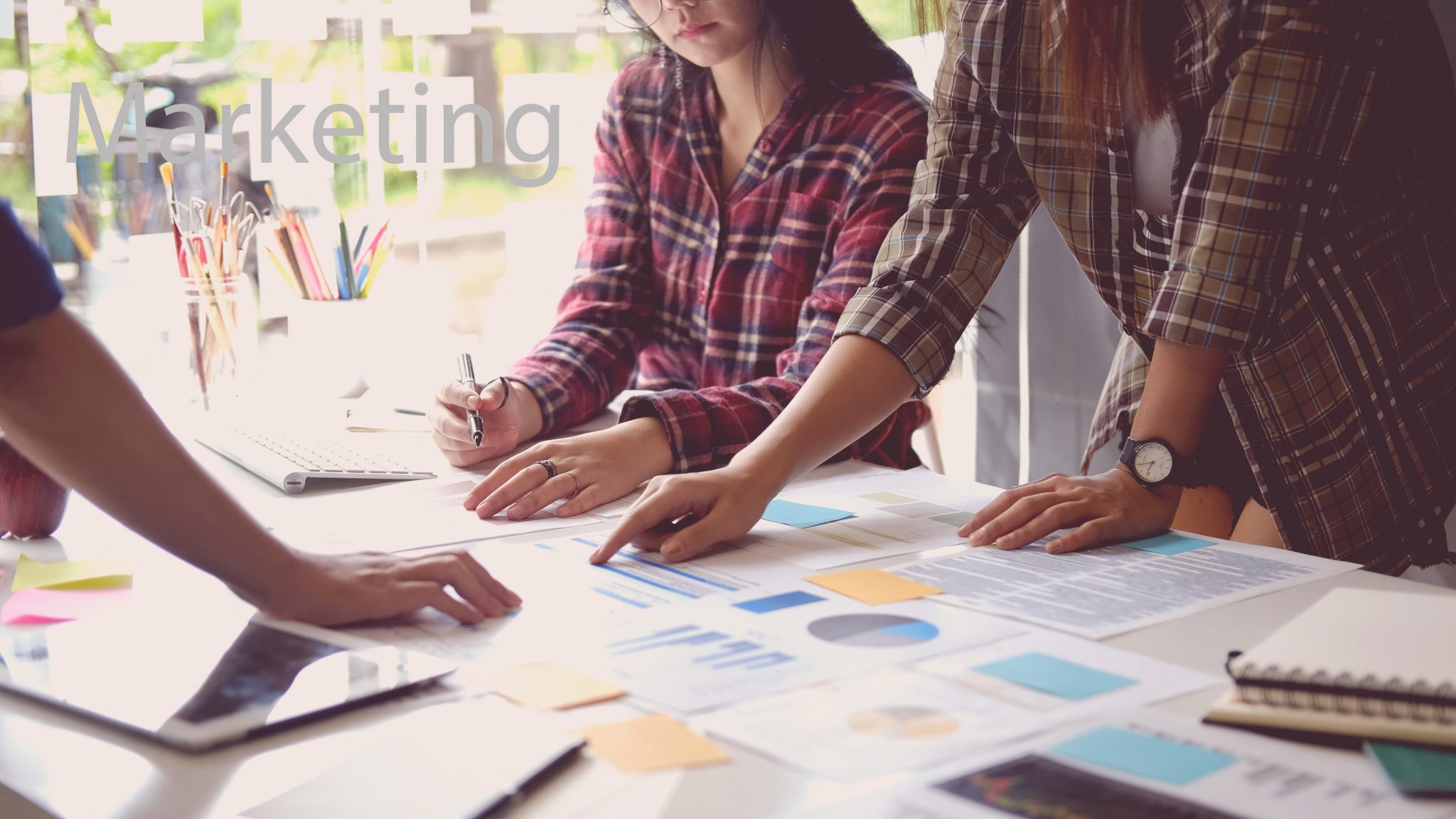
Firms routinely use choice-based conjoint (CBC) data to estimate consumers’ heterogeneous preferences. Since the amount of information elicited from each respondent is often limited, effective information pooling across respondents is critical for accurate CBC estimation. In this paper, we propose a novel affine-subspace shrinkage approach to pooling information in CBC estimation. Our approach, formulated as a simple and efficient convex optimization problem, models preference heterogeneity by shrinking the individual-level partworth estimates toward an affine subspace of the partworth space, which itself is selected as part of the estimation. Using an extensive CBC simulation experiment and two field CBC data sets, we show that our model outperforms a strong multitask learning model, and it performs comparably to a hierarchical Bayes model with a Dirichlet process prior which requires a considerably more sophisticated solution algorithm.