An Integrated Deep Learning Framework for Real-Time Air Pollution Control
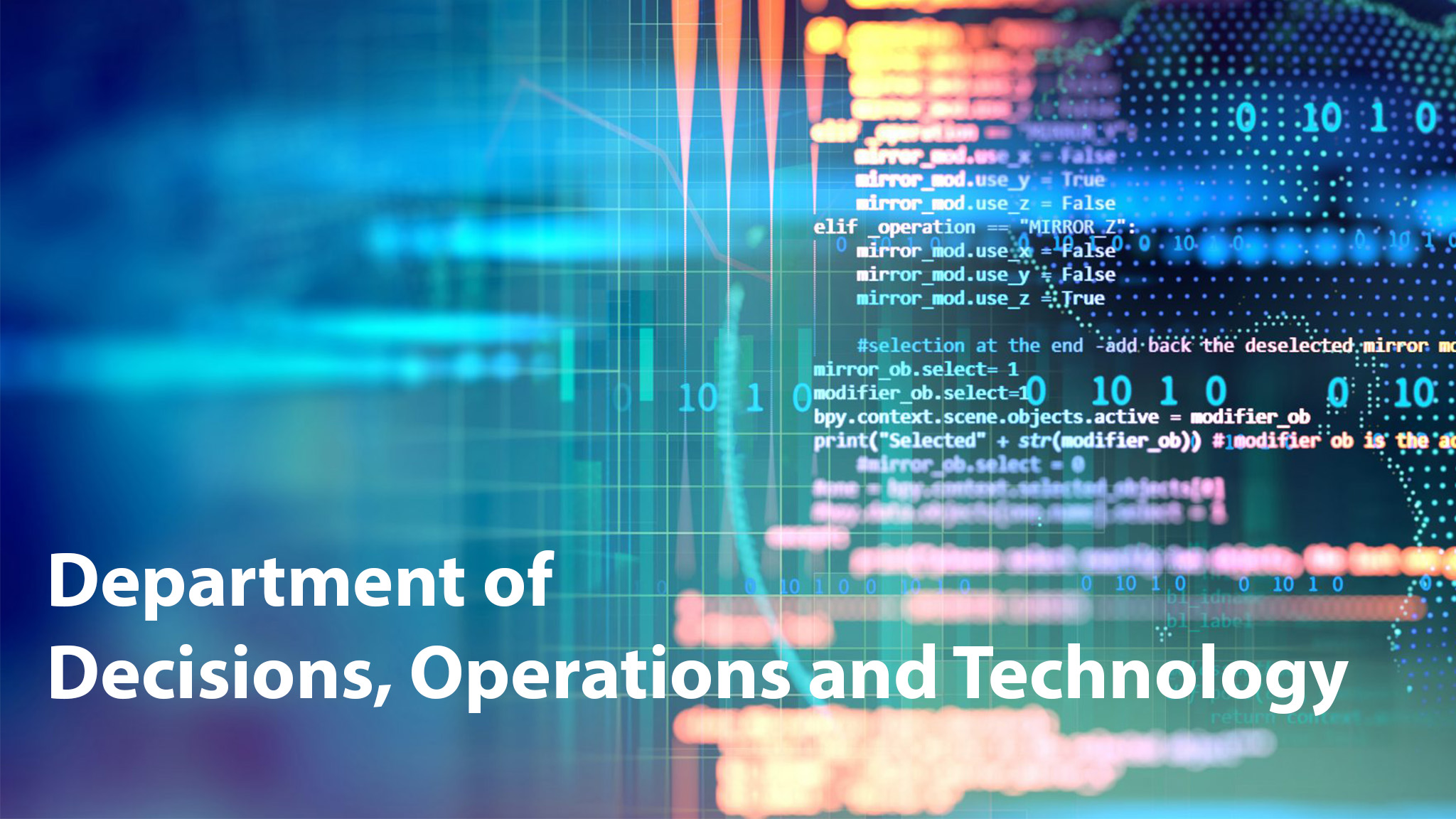
In an era marked by escalating environmental concerns and stringent regulations, various sectors, including manufacturing, energy, and electronics, are increasingly adopting advanced technological solutions for air pollution. We design an innovative deep learning-based Integrated Neural Network (INN) framework tailored for real-time air pollution monitoring and control, with an implementation on optimizing electrostatic precipitators (ESP) in power plants. This architecture furnishes system operators with precise information, enables dynamic and effective operational choices, and facilitates the transition to AI-powered control mechanisms. Utilizing second-by-second data related to air quality from a coal-fired power plant, our model adeptly identifies fluctuation patterns and outperforms the reference persistence model across 1–3 minute concentration interval forecasts on out-of-sample data, registering a remarkable 14–16\% reduction in mean absolute errors (MAE). With our partner power plant as a testament in practice, our INN architecture champions the AI-driven optimal control of ESP systems. The tangible benefits of our framework adoption extend from operational efficiency to profitability. Specifically, our collaboration with a 2-Gigawatt coal-fired power plant translates to financial gains amounting to millions of dollars yearly, complemented by annual carbon emission reduction of thousands of tons. Our design creates an IT artifact and demonstrates the potential of AI technologies’ value in environmental protection.