Data-driven Conditional Robust Optimization
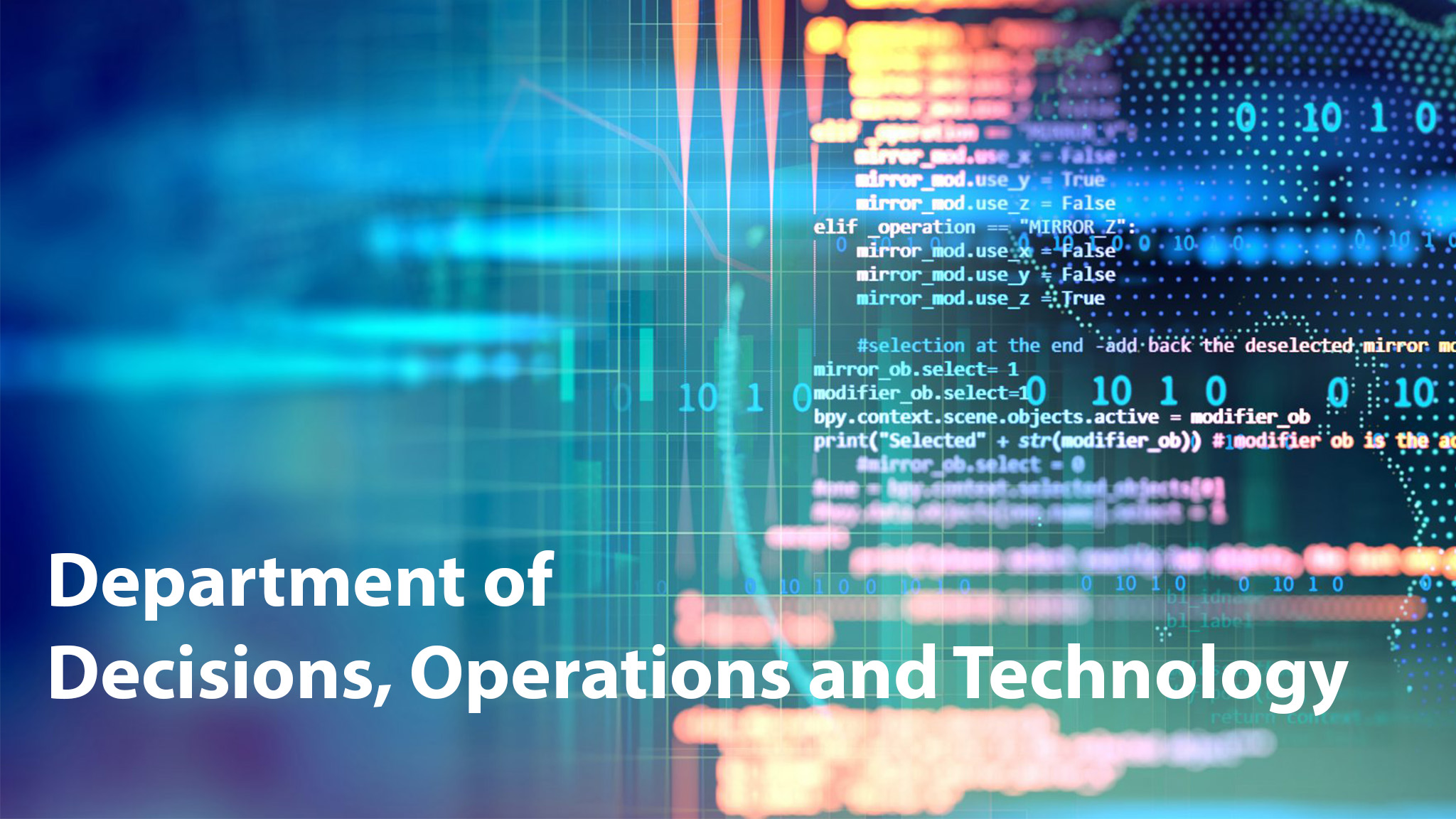
Conditional Robust Optimization (CRO) is a decision-making framework that blends the flexibility of robust optimization (RO) with the ability to incorporate additional information regarding the structure of uncertainty. This approach solves the RO problem where the uncertainty set structure adapts to account for the most recent information provided by a set of covariates. In this presentation, we will introduce two data-driven approaches to CRO: a sequential predict-then-optimize method and an integrated end-to-end method. We will also show how hypothesis testing can be integrated to the training in order to improve the quality of conditional coverage of the produced uncertainty sets.