Extracting Efficiency from Chaos: Rider Behavior, Design and Performance of Dockless Bike Sharing Systems
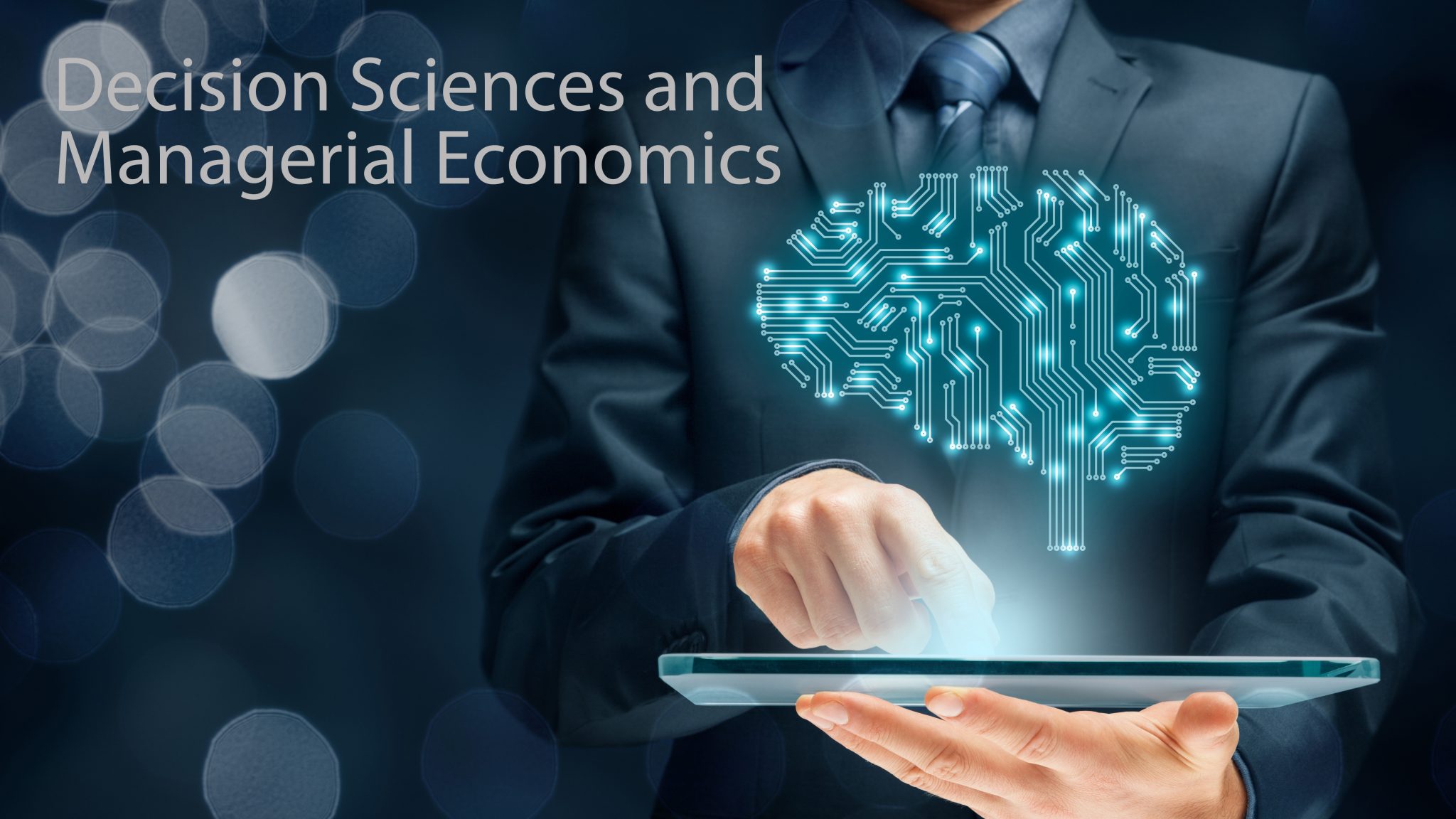
In recent years, urban bike-sharing systems developed in two main forms: Dock-based systems that rely on fixed location docks to park at and pick up bicycles from, and Dockless systems which allow users to pick up and drop off bikes anywhere. By eliminating reliance on fixed docks, dockless systems provide significantly improved mobility and convenience to riders, however they have downsides such as urban clutter and bike pile-ups that lead to under utilization of bikes and cost inefficiencies. In this paper we empirically analyze riders’ economic incentives in such a system and explore how to improve the efficiency of this business model. Specifically, we aim to answer the following questions: (i) What is the impact of the number of bicycles in the system on efficiency? (ii) How can bike relocation be best used to improve utilization? (iii) How do the efficiency of dockless and dock-based systems compare? We build a microeconomic model of user decision making in a dockless bike sharing system. We then use this model together with transaction level data from a major dockless bike-sharing firm to structurally estimate the customer utility and demand parameters. Using this estimation in counterfactual analysis, we find that the company can decrease the bicycle fleet size by 40% while maintaining 90% of transactions, leading to estimated savings of $6.5 Million. We further find that a spatial bicycle rebalancing system based on our customer utility model can improve daily transactions by approximately 19%. We also demonstrate that without bicycle redistribution, a smartly designed dock-based system can significantly outperform a dockless system. Our approach provides a predictive economic model that allows companies to estimate not only transactions, but also time and location of lost potential demand, which can be used to make targeted improvements to the geographic bike distribution. It also allows managers to fine tune bicycle fleet sizes and spatial rebalancing parameters. Further, our structural demand modeling can be used to improve the efficiency of dock-based systems by helping with targeted dock location decisions.