Identification-robust inference for the LATE with high-dimensional covariates
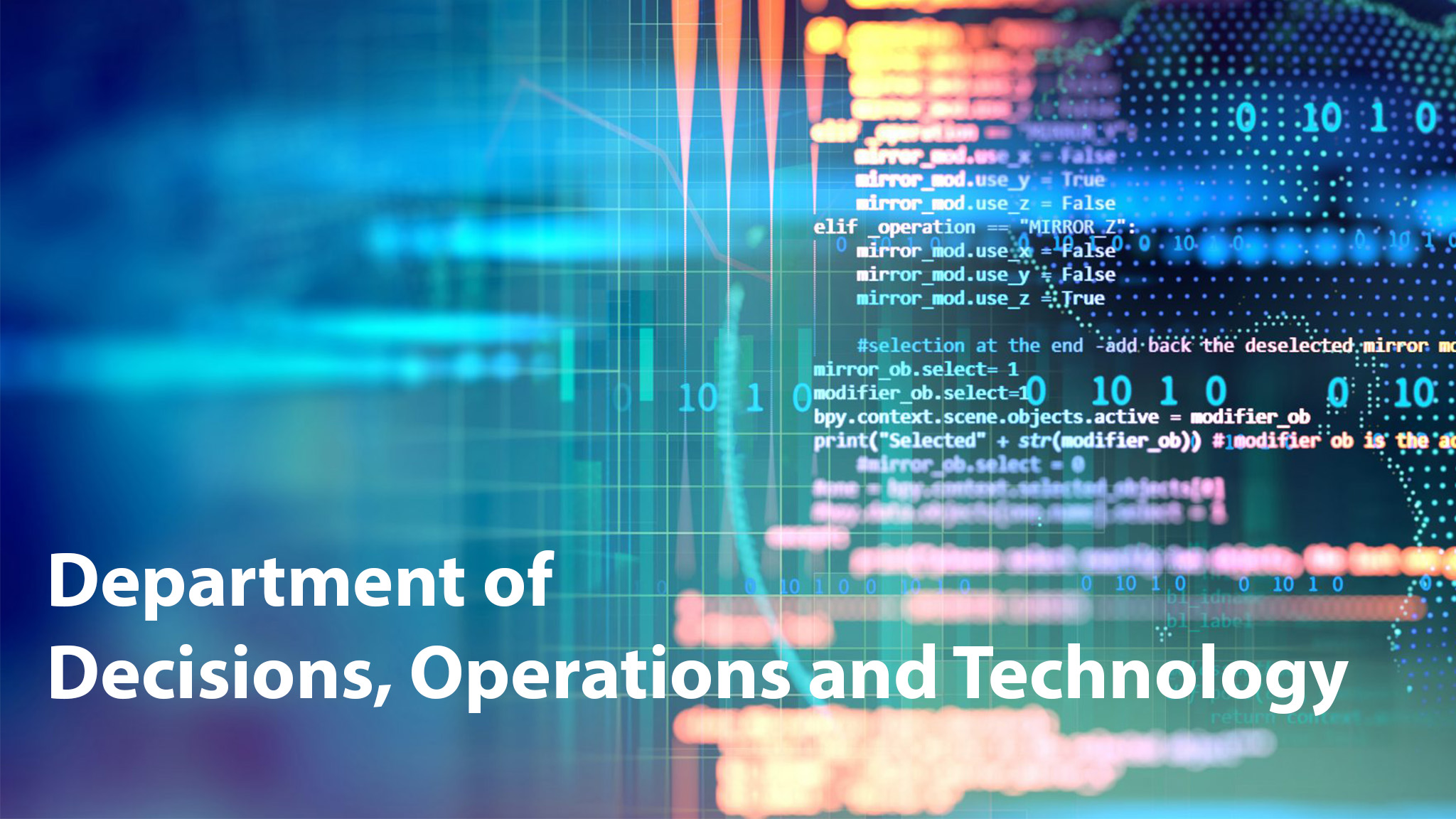
This paper presents an inference method for the local average treatment effect (LATE) in the presence of high-dimensional covariates, irrespective of the strength of identification. We propose a novel high-dimensional conditional test statistic with uniformly correct asymptotic size. We provide an easy-to-implement algorithm to infer the high-dimensional LATE by inverting our test statistic and employing the double/debiased machine learning method. Simulations indicate that our test is robust against both weak identification and high dimensionality concerning size control and power performance, outperforming other conventional tests. Applying the proposed method to railroad and population data to study the effect of railroad access on urban population growth, we observe that our methodology yields confidence intervals that are 49% to 92% shorter than conventional results, depending on specifications.