Learning While Repositioning in On-Demand Vehicle Sharing Systems
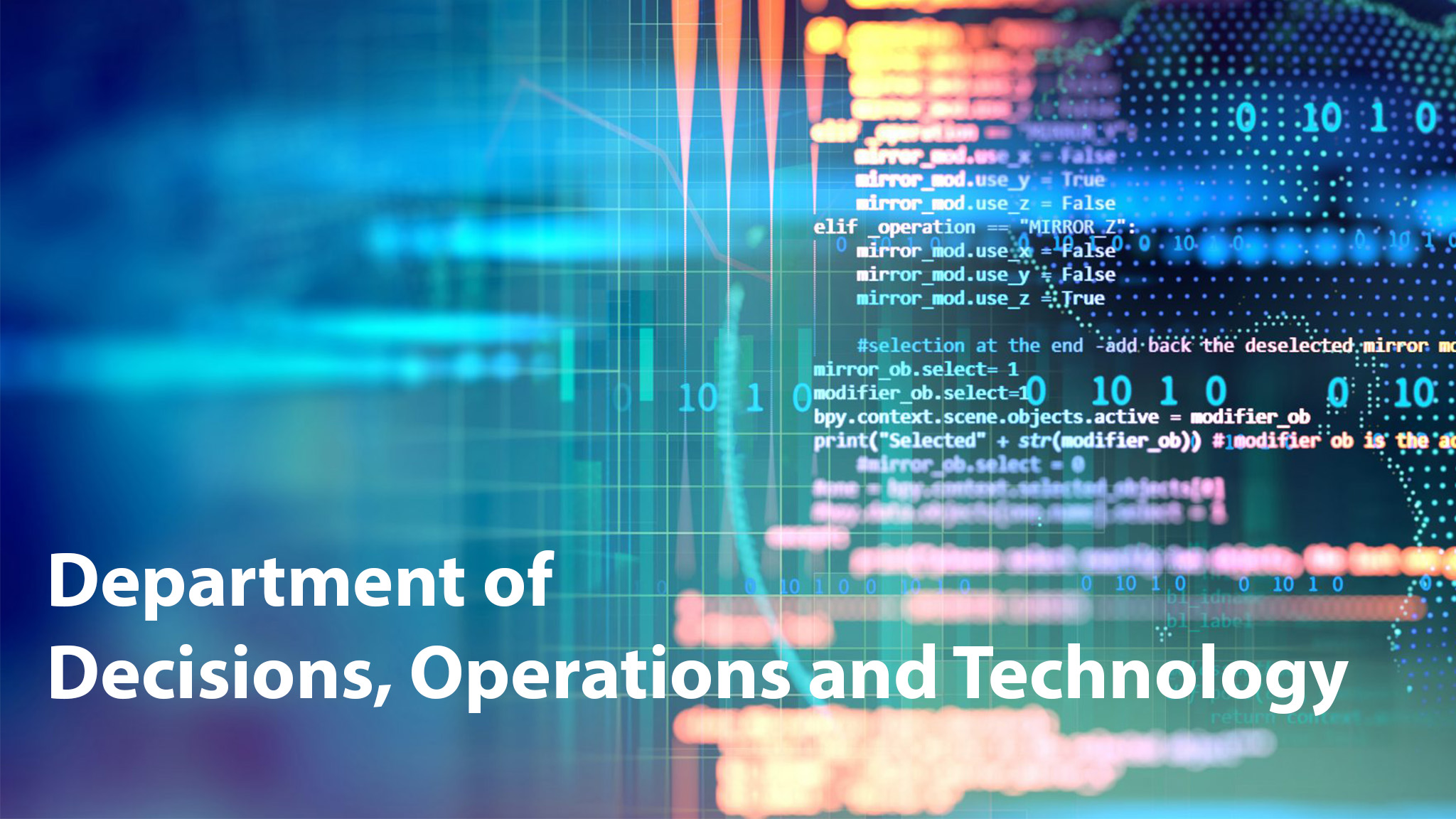
We consider the vehicle repositioning problem for a one-way, on-demand vehicle sharing service with a fixed number of rental units distributed across the network. Due to uncertainty in both customer arrivals and vehicle returns, the service provider needs to periodically reposition the vehicles to match the supply with the demand while minimizing the total costs of repositioning labor and lost sales. The optimal repositioning policy under a general multi-location network is intractable without knowing the optimal value function. We define the best base-stock repositioning policy as a generalization of the popular inventory control policy to the vehicle repositioning problem, and we establish its asymptotic optimality in two different limiting regimes under general network structures. We provide reformulations to solve the offline problem of finding the best base-stock policy, and prove that the offline solution has favorable generalization properties. In the online setting, we develop an Online Gradient Repositioning algorithm utilizing only censored demand and, under a mild cost structure condition, we prove that it achieves an optimal regret, which matches the regret lower bound. Our online algorithm is designed by carefully decomposing the cumulative costs and formulating a linear programming problem whose dual solution serves as the gradient. Moreover, we provide and discuss three other online algorithms to elucidate the inherent challenges of learning while repositioning, and our analysis offers new insights into learning with censored data in networks. Numerical experiments illustrate the effectiveness of our proposed approach.