Machine Learning versus Economic Restrictions: Evidence from Stock Return Predictability
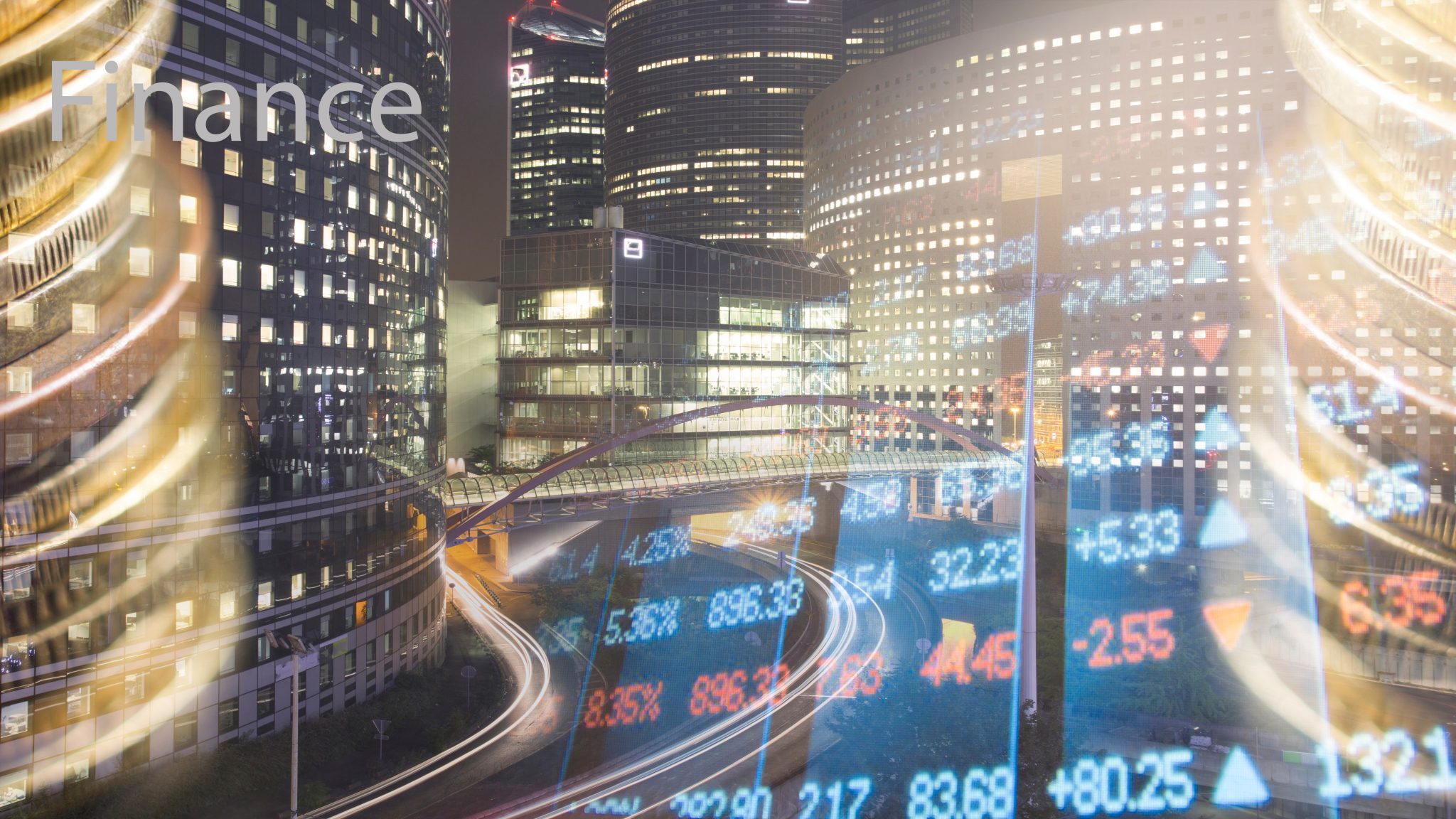
This paper shows that machine learning methods often fail to clear standard economic restrictions. Machine learning-based investments extract profitability primarily from difficult-to-arbitrage stocks and during alleviated limits-to-arbitrage market states. Value-weighting returns and excluding microcaps or distressed stocks considerably attenuate profitability. Performance further deteriorates in the presence of trading costs due to high turnover or extreme positions in the tangency portfolio implied by the pricing kernel. Despite their opaque nature, machine learning methods identify mispriced stocks consistent with most anomalies. Beyond economic restrictions, deep learning signals are profitable in long positions, remain viable in recent years, and command low downside risk.