Measuring Readability with Language Predictability: A Large Language Model Approach
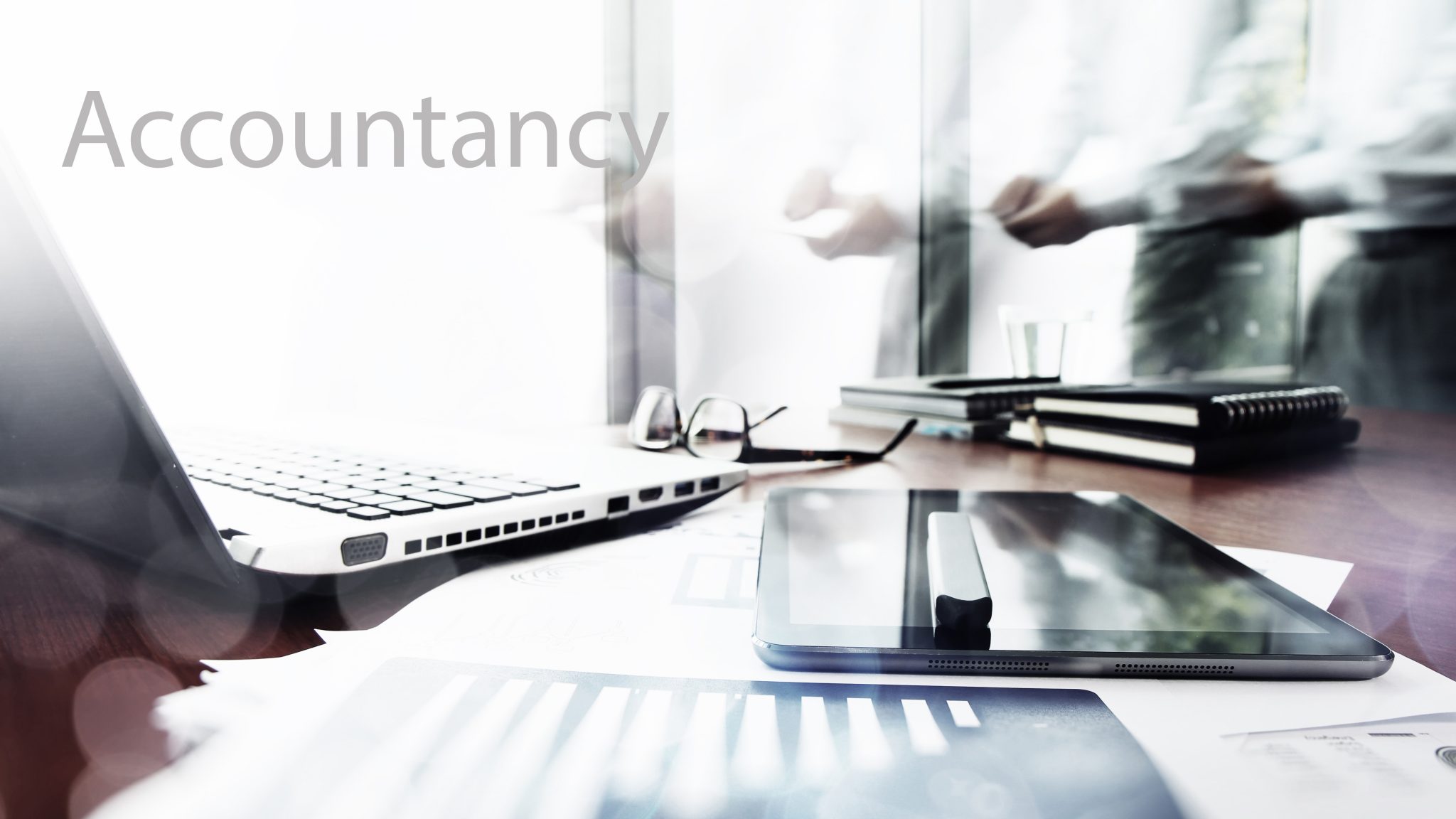
Abstract
We propose a new readability measure, the language predictability score (LPS), to assess the processing costs associated with comprehending corporate disclosure text. Our measure has strong theoretical roots and experimental support in psycholinguistics and cognitive science. Unlike the three most commonly used readability measures (i.e., the Fog index, the Bog index, and file size), the LPS measures readability based on the context of words and the target audience of the text. We use the large language models GPT-2 and BERT—which, after pre-training and fine-tuning, imitate the language ability of investors—to estimate the LPSs of a large sample of management discussion and analysis (MD&As) of annual reports. In validity tests, we show that the LPS identifies incoherent text as less readable and boilerplate content as more readable. In the main tests, we show that, after controlling for firm fixed effects, the LPSs of MD&As are significantly associated with post-filing stock return volatility as well as dispersion and accuracy in analysts’ earnings forecasts and that the LPS outperforms the Fog index, the Bog index, and file size in explaining analysts’ processing costs.
For enquiries, please contact Ms. Heidi Lam at heidilam@cuhk.edu.hk.