Nonprogressive Diffusion on Social Networks: Approximation and Applications
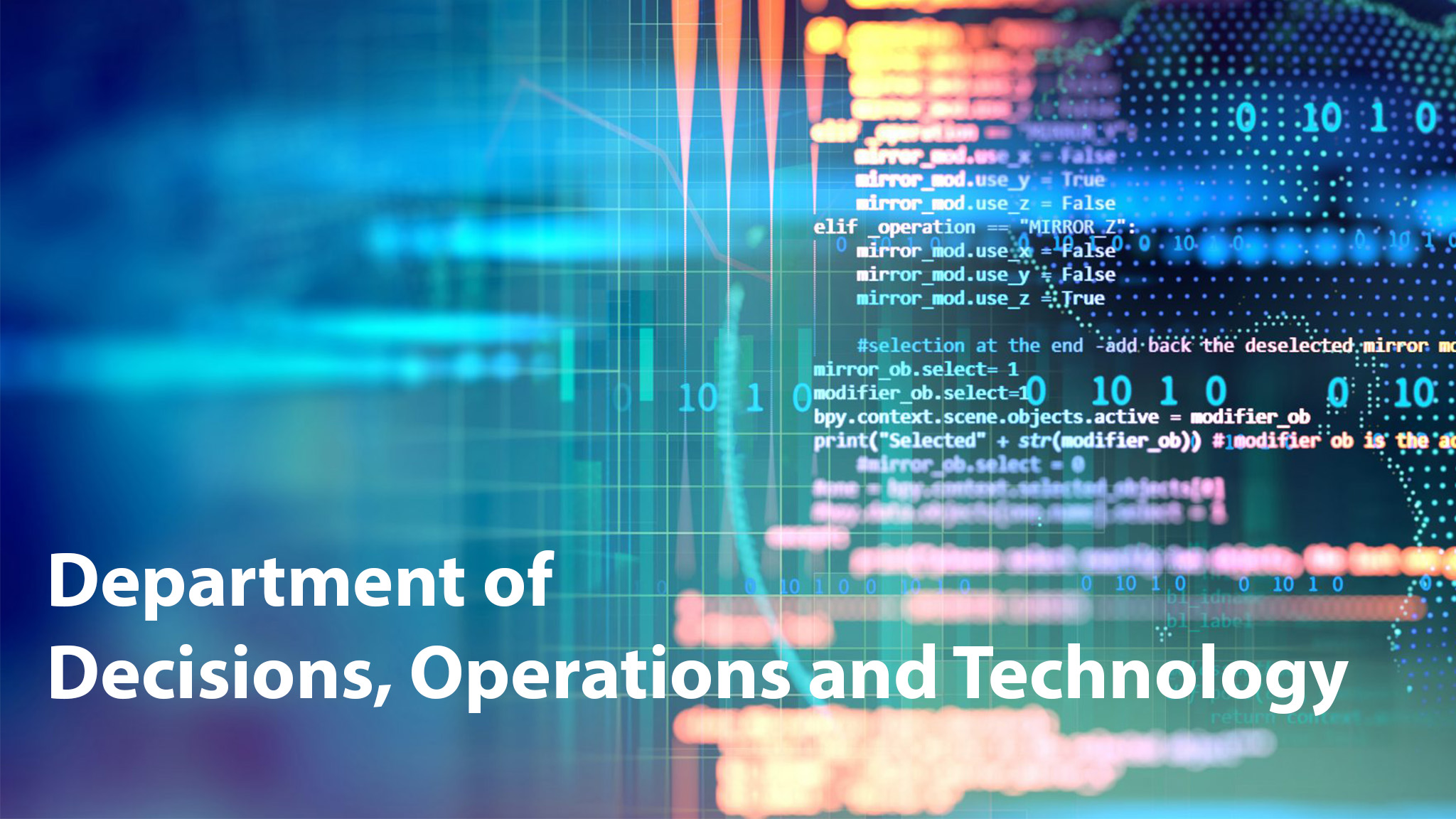
Nonprogressive diffusion describes the spread of behavior on a social network, where agents are allowed to reverse their decisions as time evolves. It has a wide variety of applications in service adoption, opinion formation, epidemiology, etc. To offer an efficient framework for evaluating and optimizing nonprogressive diffusion, we introduce a comprehensive model and a Fixed-Point Approximation (FPA) scheme. This approximation scheme admits both a theoretical guarantee and computational efficiency. We establish that the approximation error is inherently related to the network structure, and derive order-optimal bounds for the error using two novel metrics of network characteristics. We show that the FPA scheme is most powerful for dense and large networks that are generally prohibitive to analyze by simulation. Taking the widely studied influence maximization and pricing problems on a social network as examples, we further illustrate the broad applications of our FPA scheme. Finally, we conduct comprehensive numerical studies with synthetic and real-world networks. In real networks, the FPA scheme shows 70-230 times more speed up in computation time than simulation while achieving a mean absolute percentage error of less than 3.48%. Moreover, our proposed two network metrics are reliable indicators of the FPA scheme’s performance.