Online Algorithms for Correlated Arrivals
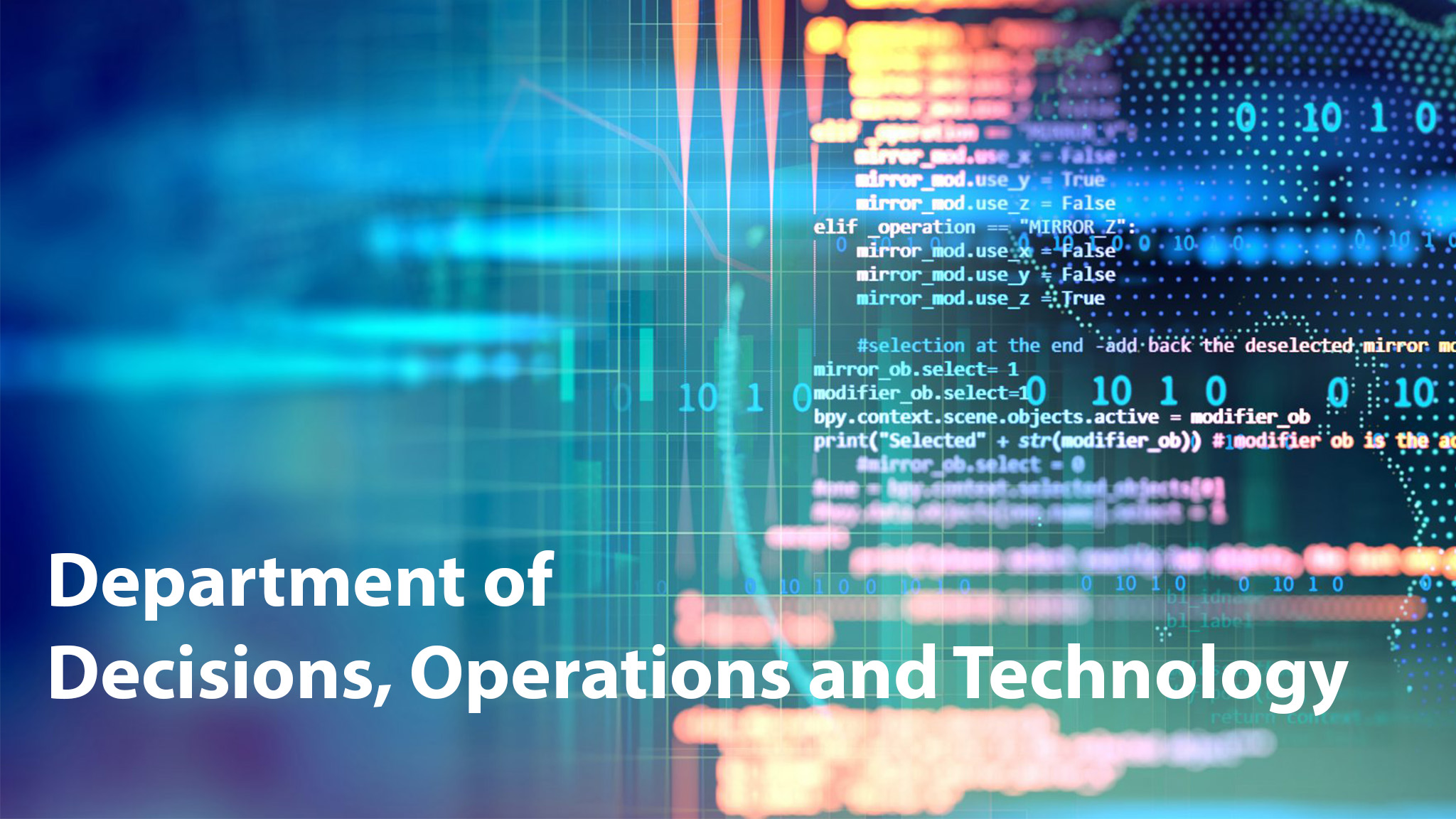
Online algorithms are ubiquitous in e-commerce systems, where inventory, fulfillment, and assortment decisions must be committed to in real-time without knowing future demand. For many of these problems, there is a lack of decision-making approaches that are aware of correlations in demand over time. This is partly due to the challenge of model selection, but even given an explicit model of correlated demand, it is unclear how online decisions should be prescribed.
We present two new approaches that overcome these challenges by implicitly capturing the correlations in demand arrivals over time. First, we present a non-parametric framework that models only the distribution of the total arrivals of each “type”, and then by making assumptions on how these arrivals are interleaved, leads to correlation-aware online decisions. Second, we discuss a model-free “reinforcement learning” approach that directly optimizes for average performance over a collection of past (correlated) arrival sequences, while restricting to a carefully curated class of policies. We discuss the benefits of each approach, both of which leverage the ability to evaluate counterfactual policy performance in inventory and fulfillment problems. Finally, we discuss details of the first approach specifically for the online matching (fulfillment) problem, which is based on joint work with Ali Aouad (London Business School -> MIT).