Online Personalized Assortment Optimization in A Big Data Regime
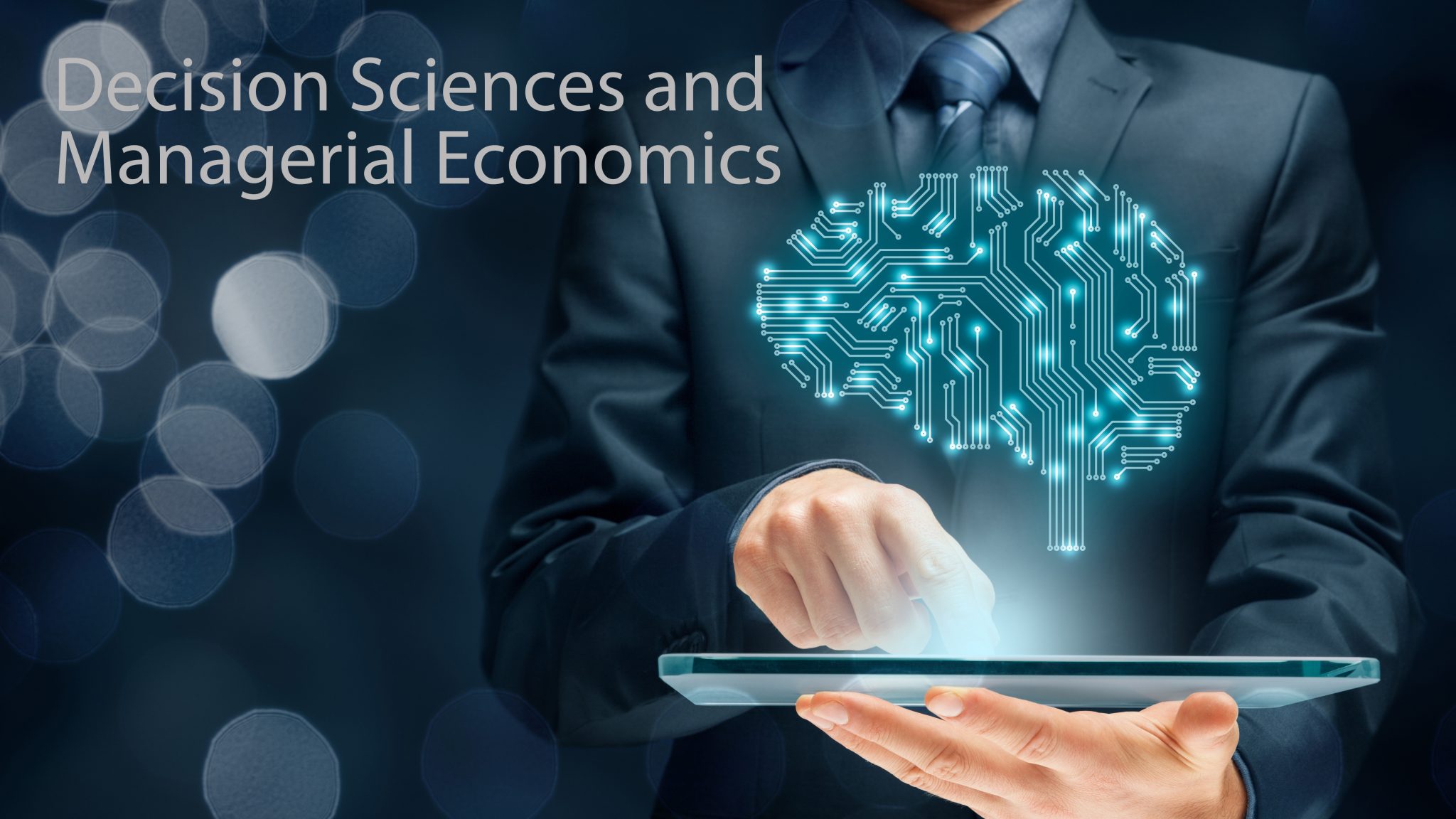
We consider an online personalized assortment optimization problem where customers arrive sequentially and make their choices (e.g., click an ad, purchase a product) following the multinomial logit (MNL) model with unknown parameters. Utilizing customer’s personal information, the firm makes an assortment decision tailored for the individual customer’s preference. This problem typically faces the challenge of “big data”, i.e., a huge amount of customers arrive within a short period of time and their personal information vectors are typically high dimensional. To tackle this challenge, we develop an algorithm which makes assortment recommendations to maximize expected total revenue while concurrently learning the demand. This algorithm combines the method of random projection (for dimension reduction to tackle high dimensionality issue) and an online convex optimization procedure (for parameter estimation to tackle huge number of data samples). The theoretical performance for our algorithm in terms of regret is derived, and numerical experiments using real data demonstrate that it performs very well compared with several benchmarks.