Optimal Bundling Design: An Empirical Investigation
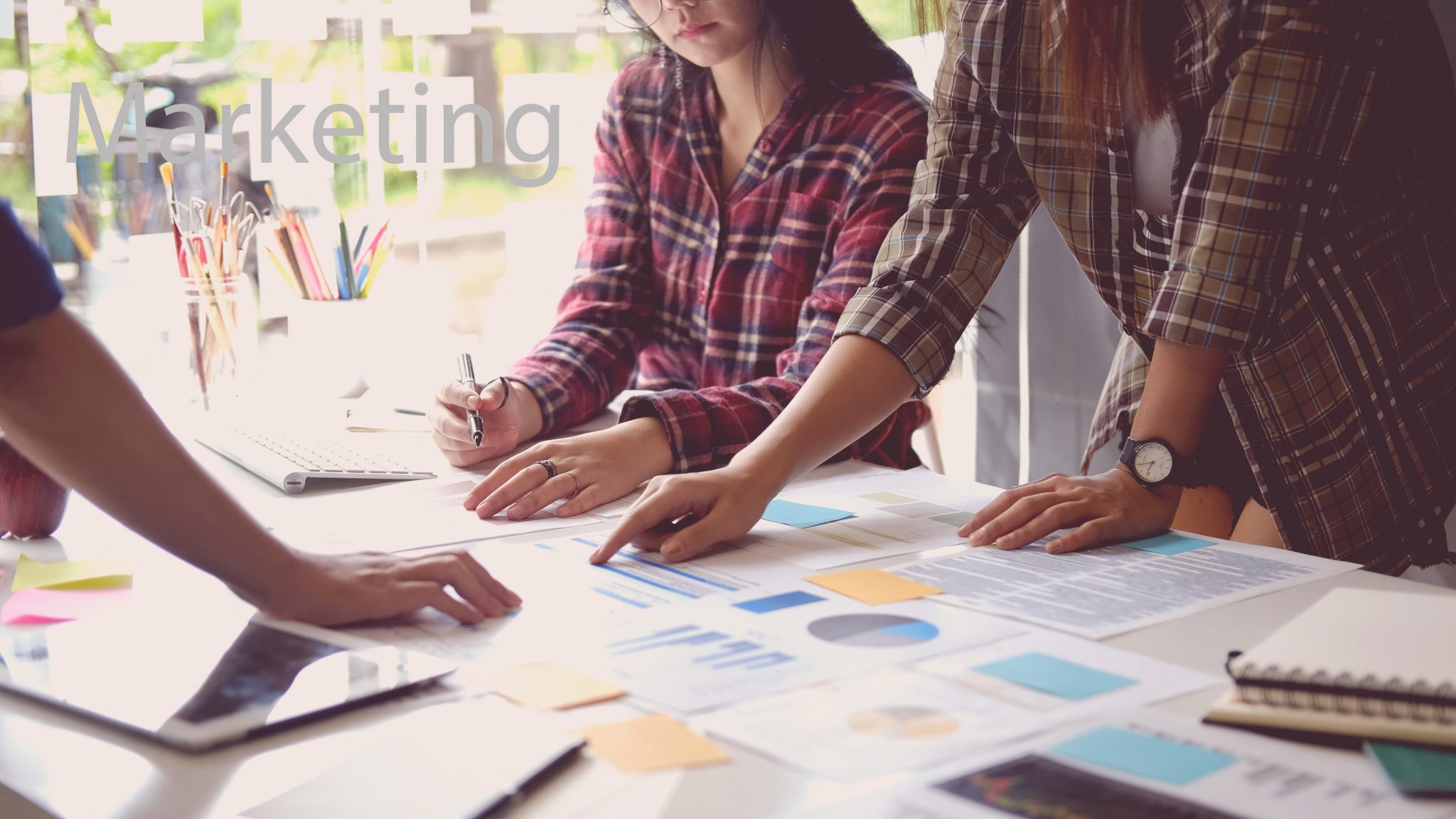
We empirically investigate the effects of service bundling, as a form of the second-degree price discrimination, on the subscription and usage behaviors of consumers. Our goal is to help firms design optimal bundles, which involve the number of bundles and the size and price of each bundle, that will maximize their profit. In our setting, service bundles have to be purchased first and consumed later, and firms can simultaneously offer bundle and linear pricing. Consequently, bundle subscribers may under- or over-use the service offerings, creating additional benefit for firms.
Utilizing data from a premium gym that offers personal training sessions in the US, we develop a structural model of consumer subscription and consumption choices under service bundling. Our model flexibly captures rich consumer heterogeneities in exercise preferences, price sensitivities and demand uncertainties, using a Gaussian Mixture distribution specification. Based on the estimation results, we run a series of counterfactuals to explore the optimal bundling design of the gym. We leverage the neural network algorithm in the computation and show its advantages over the standard numerical search algorithm when the counterfactuals are associated with a large number of decision variables under rich consumer heterogeneities. Our research extends the theoretical studies of optimal bundles in the previous literature, and provides empirical insights and practical implications for firms who seek to capitalize on the full potential of bundle offerings.
Keywords: Second-Degree Price Discrimination, Optimal Bundles, Consumer Behavior, Structural Modeling, Neural Networks, Gaussian Mixture Distribution, Consumption Uncertainty