Queueing Analytics: Machine Learning, Causal Queueing, and SiMLQ for Data Driven Simulation
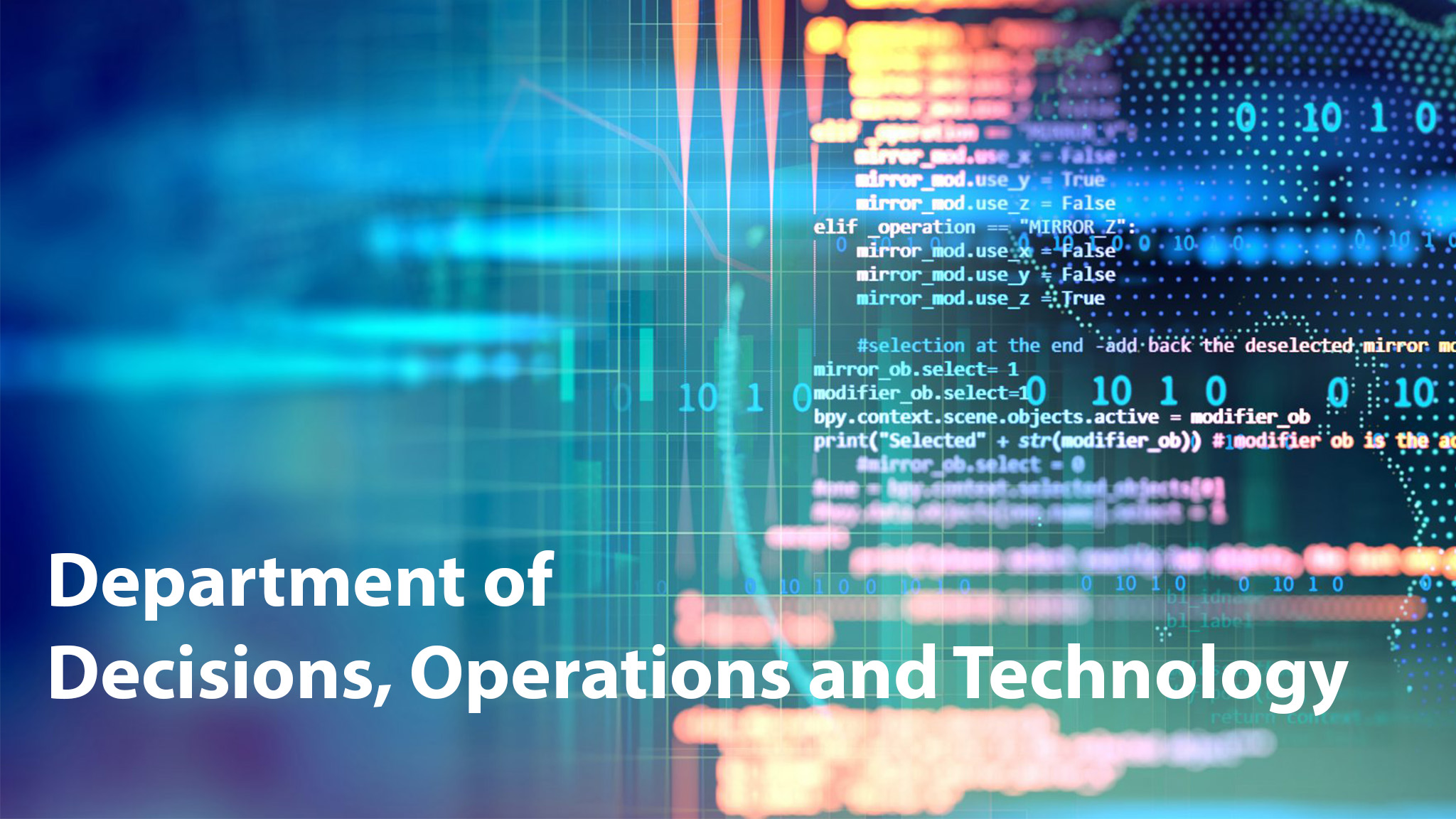
The objective of this talk is to expose researchers to the vast possibilities of using modern machinery and data for implementing effective management analytics for processes that can be modeled as queueing systems. Such process are ubiquitous in modern economies, e.g., customers waiting to service, inventory waiting for processing/transportation, payments and invoices waiting to be generated/cleared, computing tasks waiting for resources. I will thus discuss recent developments in queueing analysis based on several papers.
We will start by defining management analytics along descriptive, predictive, comparative, i.e., comparing performance indicators under different interventions, and prescriptive analytics dimensions. We then shortly discuss ML solution for a G/G/1 based upon [1] and its extension to G(t)/G/1 based on [2].
Our main focus would be on causal queueing models, based upon [3]. Not many organizations have queueing theorists (QTs) in their staff, but many organizations employ well trained Data Scientist (DS). Can DS use data to provide accurate comparative analytics without expertise in queueing? We suggest a data-driven representation of system building blocks to create a non-queueing simulator without prior knowledge of the system. We show that this approach is effective in comparative analytics, when analyzing expected waits for an GI/M/1 with speed-ups. We first demonstrate that DS can successfully refine the parent sets of queueing variables from data using an off-the-shelf algorithm (even under a moderate sample size). We then use machine learning to estimate the causal structure in this queue, e.g., the Lindley’s Recursion and use the G-computation to derive inference results of counterfactual interventions. For the GI/M/1 with speed-ups, we compare the performance of estimates obtained by a QT, who uses data driven estimates for the primitives of the queue, with those made by a DS that uses either parametric (where inter-arrival and service time distributions are known) or nonparametric (where both distributions are unknown) estimators. We find that the errors of the DS that requires no knowledge of the system’s dynamic and its features and these of the QT (which requires this knowledge) are comparable. Our results suggest that the DS approach would be effective for practical setting- where even experts QTs cannot provide closed-form results.
We will finish with a short demo of SiMLQ. SiMLQ software uses Machine Learning to automate the visualization, Simulation, and optimization of Queueing processes. SiMLQ automatically constructs data-driven simulation models from event-log data collected by common information systems and enables users to improve processes resource management, increase efficiency, reduce cost, and manage risks. SiMLQ- from data to action.
[1] Sherzer E., Baron O., Krass D., Senderovich A., (2023) Supervised Machine Learning for Solving General Queueing Systems INFORMS Journal on Computing (Forthcoming)
[2] Sherzer E., Baron O., Krass D. Reshef, H., Senderovich A., Approximating G(t)/GI/1 queues with deep learning
[3] Baron, O., Krass, D., van der Laan, M., Senderovich, A,. Xu Z. Queueing Causal Models: Comparative Analytics in Service Systems.