Segmenting Consumer Location-Product Preferences for Assortment Localization
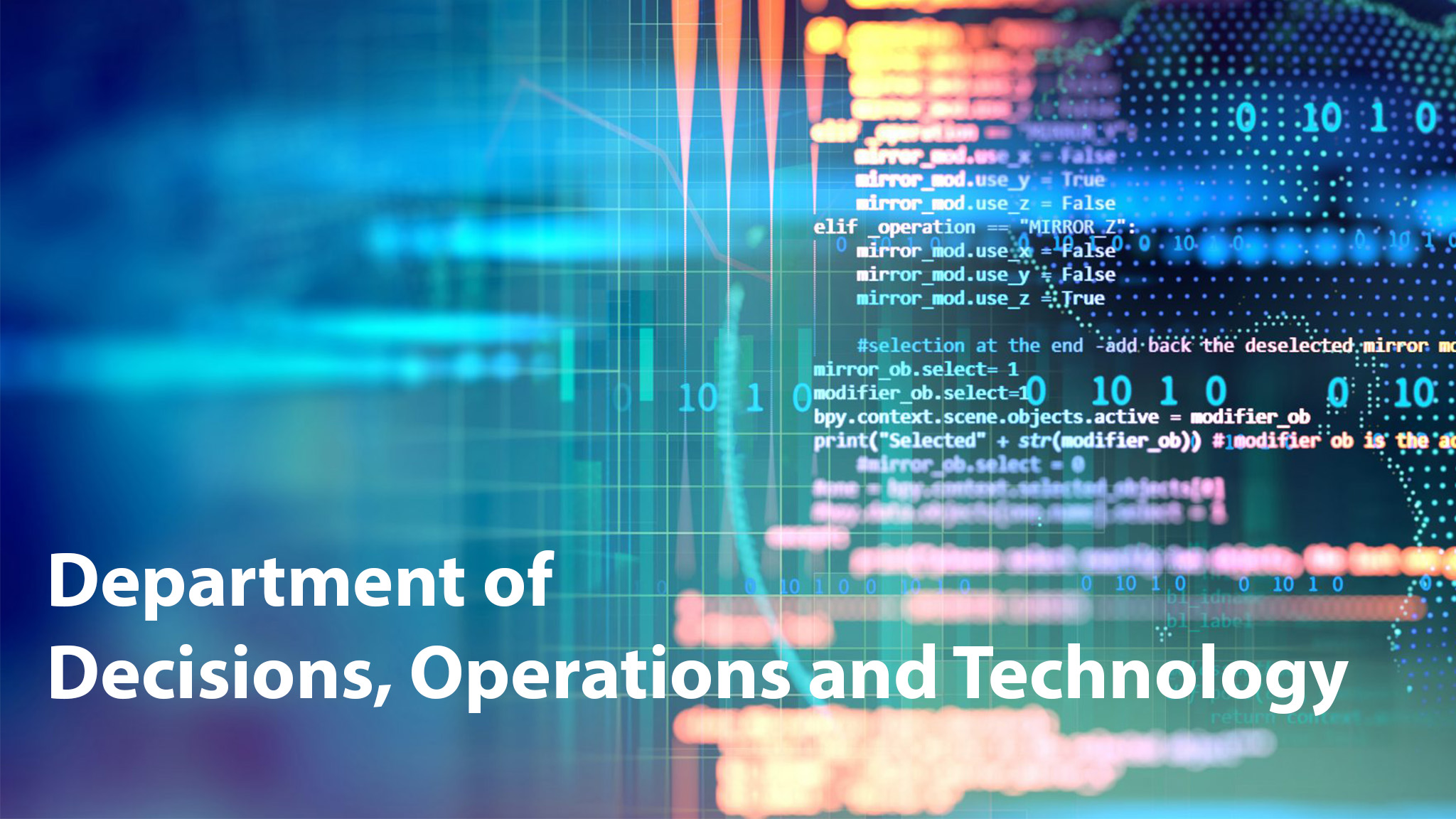
When managing multiple stores in the same marketplace, retailers need to select store locations and localize product assortments to reflect the heterogeneous demand preferences across communities. This paper introduces a customer-centric data-driven framework for interpretable, flexible, and scalable localized assortment optimization. The framework involves a dynamic system, called the dual Poisson Dynamic System with Multilayer Factorization (dPDS-MF), for panel data on product assortments and individual consumer purchases across vending locations, The dPDS-MF can help retailers automatically profile different consumer segments driven by store visiting preferences, measure the relationships across store locations, and estimate the product preferences for each consumer segment simultaneously. The framework also involves an automated decision model for optimizing localized assortments, based on a trained dPDS-MF. We apply our proposed framework in the retail vending market in major train stations in Japan. We showcase the face validity of the model outputs and demonstrate the value of the dPDS-MF for guiding decisions on vending locations and localizing product assortments. Specifically, we show that compared with several benchmark strategies, including the nested-logit choice model, our proposed assortment strategy not only improves the expected revenue up-to 30% but also gives more meaningful localized assortment strategies.