Size substitution and fairness in assortment optimization
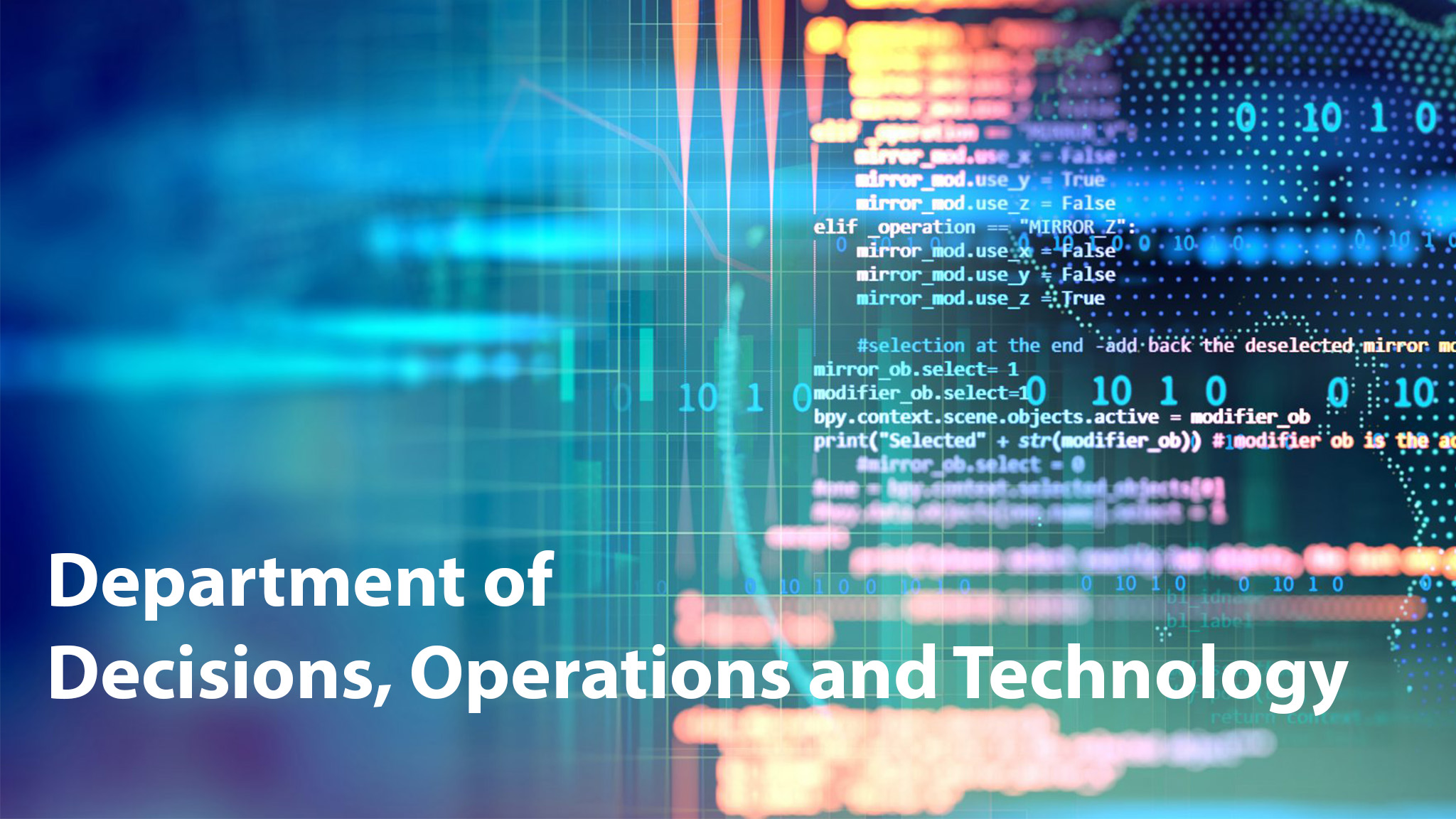
Size stockouts in online fashion retailing occur when an item is not available in one of its sizes. This could be because offering the full-size range is not optimal for the retailer or the inventory of a particular size is sold out. Size stockouts may also trigger fairness concerns among customers. In recent years, there has been substantial coverage of discrimination against plus-size customers as they cannot find the items they like in suitable sizes. We model the assortment optimization problem of an online fashion retailer subject to size substitution and fairness considerations. We capture the size substitution effect as the probability that customers switch to neighboring sizes of the same style if their target size is out of stock. We further introduce a measure of availability as a group-level metric of the probability that a customer of a size group can find an item proper for her size. We use this metric to define our unfairness index as the gap between the best and worst-off size groups. We introduce efficient algorithms to find the additive epsilon-approximate solution to our problem which may entail a randomized assortment. We calibrate our data-generating functions using a real sales dataset and then run a series of numerical simulations. Among others, we find that: in a considerable proportion of samples, with just 2% sacrifice in the expected revenue, a retailer can alleviate the unfairness in its assortment by almost 50% using our algorithms. Moreover, the number of distinct assortments a retailer must show using randomization is usually low (three or fewer,) which signifies the practicality of our solution. Finally, even with a large approximation coefficient, the gap between the upper bound for the optimal solution and the outcome of our algorithms tends to be small. This is promising as we can then develop fast algorithms without compromising too much on accuracy.