Social Learning and Polarisation in Content Platforms
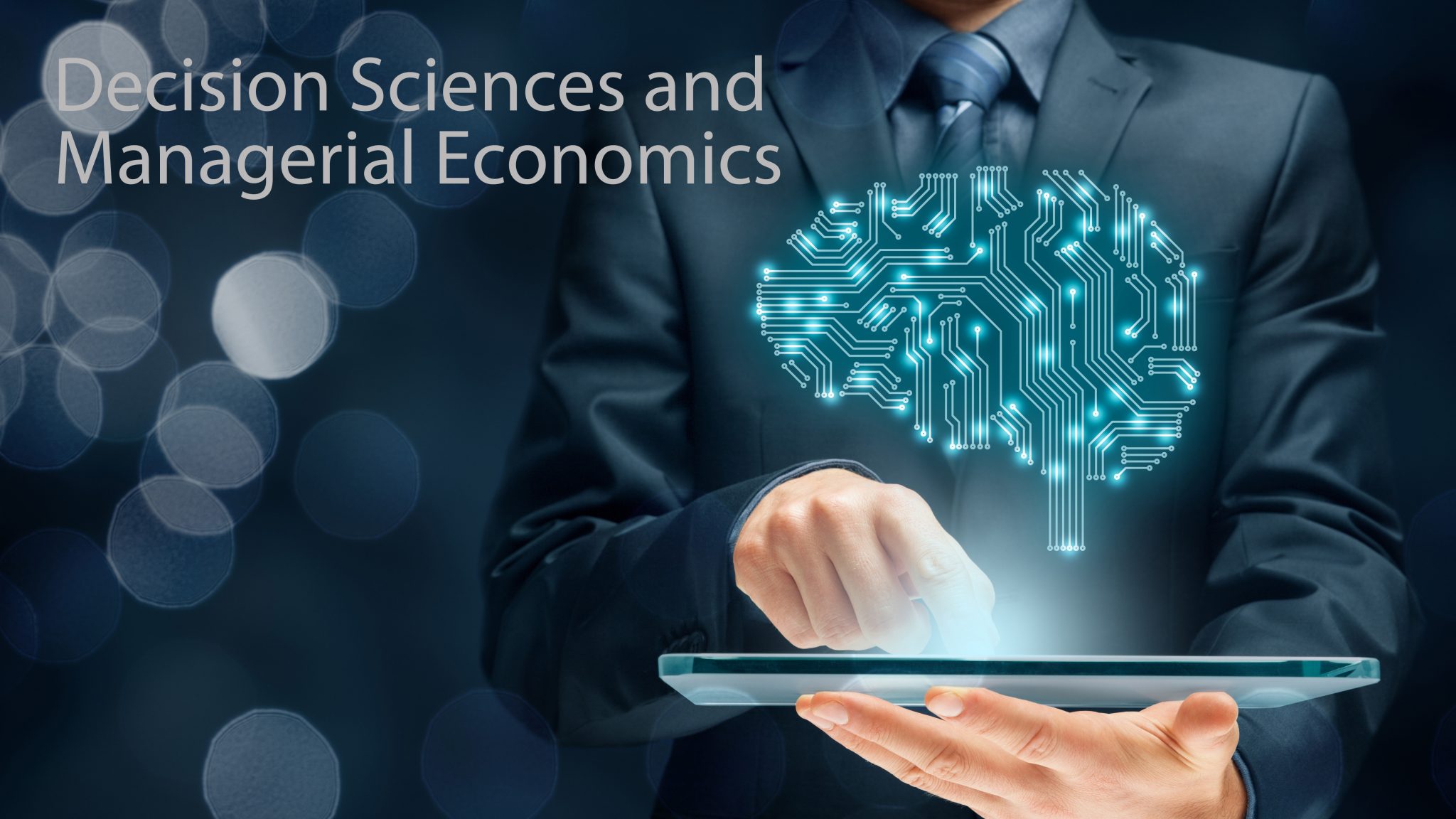
Today, a significant portion of the society consumes content posted by independent content providers on platforms (e.g., YouTube), to gather information pertaining to issues about which consumers may be polarised, i.e., have opposing beliefs. Contemporary polarising issues include COVID-19 vaccine mandates, abortion rights, and climate change, which attract strong anti- vs. pro-positions. The quality of such content is hard to determine prior to consuming it. Hence, consumers may rely on aggregate consumption metrics (e.g., number of “Views’” on YouTube) and other informative signals provided by the content platform to assess the quality of the content that either support or oppose their belief on the issue. Based on a stylised model, we find that a social learning (SL) mechanism based on aggregate consumption metrics can mislead consumers to incorrectly perceive a low-quality content to be of higher quality. The incomplete learning phenomenon aggravates as consumers become more uncertain about the distribution of population preferences. We characterise parametric regimes where the (financial) interests of the platform and the content provider are in conflict with the welfare of consumers. As a result, SL may lower the incentive for the content provider to improve the content quality and by extension, the platform would prefer to facilitate SL by displaying consumption metric to mask the underlying low quality of the content. These findings continue to hold when the platform selectively recommends the content to consumers whose belief the content caters to. Greater accuracy of a content recommendation policy improves the SL outcome, but it may also lower the incentive of the content provider to improve quality due to the formation of so-called “echo-chamber.” We also discuss broader implications of our results for quality control on content platforms.