Social Media-Driven Credit Scoring: The Predictive Value of Social Structures
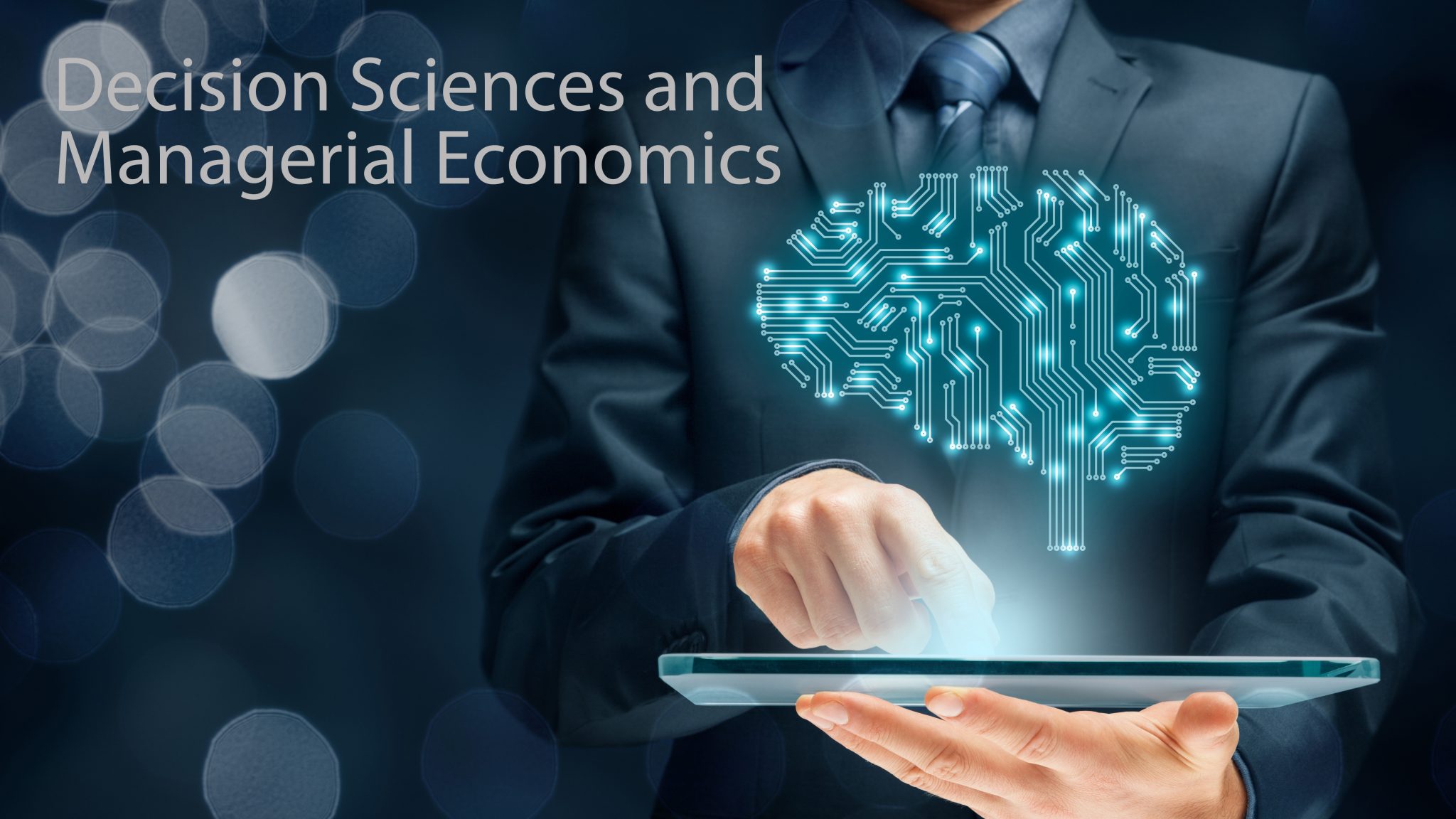
In this talk, I will first cover an overview of my research on social networks, network evolution, analytics, and media. Following, I will discuss a framework and a pipeline of projects related to Analytics, Social Networks, and Fintech. At the intersection of Fintech, analytics and AI lies an interesting area of research whereby traditional methods and approaches are not sufficient to capture the rich context, nor address appropriate data context or restrictions. I present several projects looking at credit scoring using multi-modal data including from social networks and mobile devices to reach the 2+ billion unbankables throughout the world. I discuss on-going projects using artificial intelligence to close the loop on repayment behavior. Finally, I will discuss some psychological mechanisms such as financial literary which affect borrowers’ behavior in emerging markets. Following, I will dive deep into a paper which innovates on new methods for social network-based credit scoring.
While emerging economies have seen an explosive growth of Internet population, these countries lack sophisticated credit scoring system or credit bureaus to predict creditworthiness of individuals. Leveraging the widespread adoption of social media and social network sites in emerging markets, microfinance institutions innovate on new credit scoring methods using novel data sources. In this paper, we propose a Bayesian method for social network-based credit scoring that helps to address network sparsity and data scarcity, common with ego-centric networks. Our empirical results suggest that by incorporating social network information, we can improve the creditworthiness prediction in microfinance by up to 300%. We believe that although lending to the poor without incurring high default rates is challenging, social network-based methods can be an effective approach used for developing countries that face the financial exclusion problem.