Stochastic Optimization with Decisions Truncated by Random Variables and Its Applications in Operations
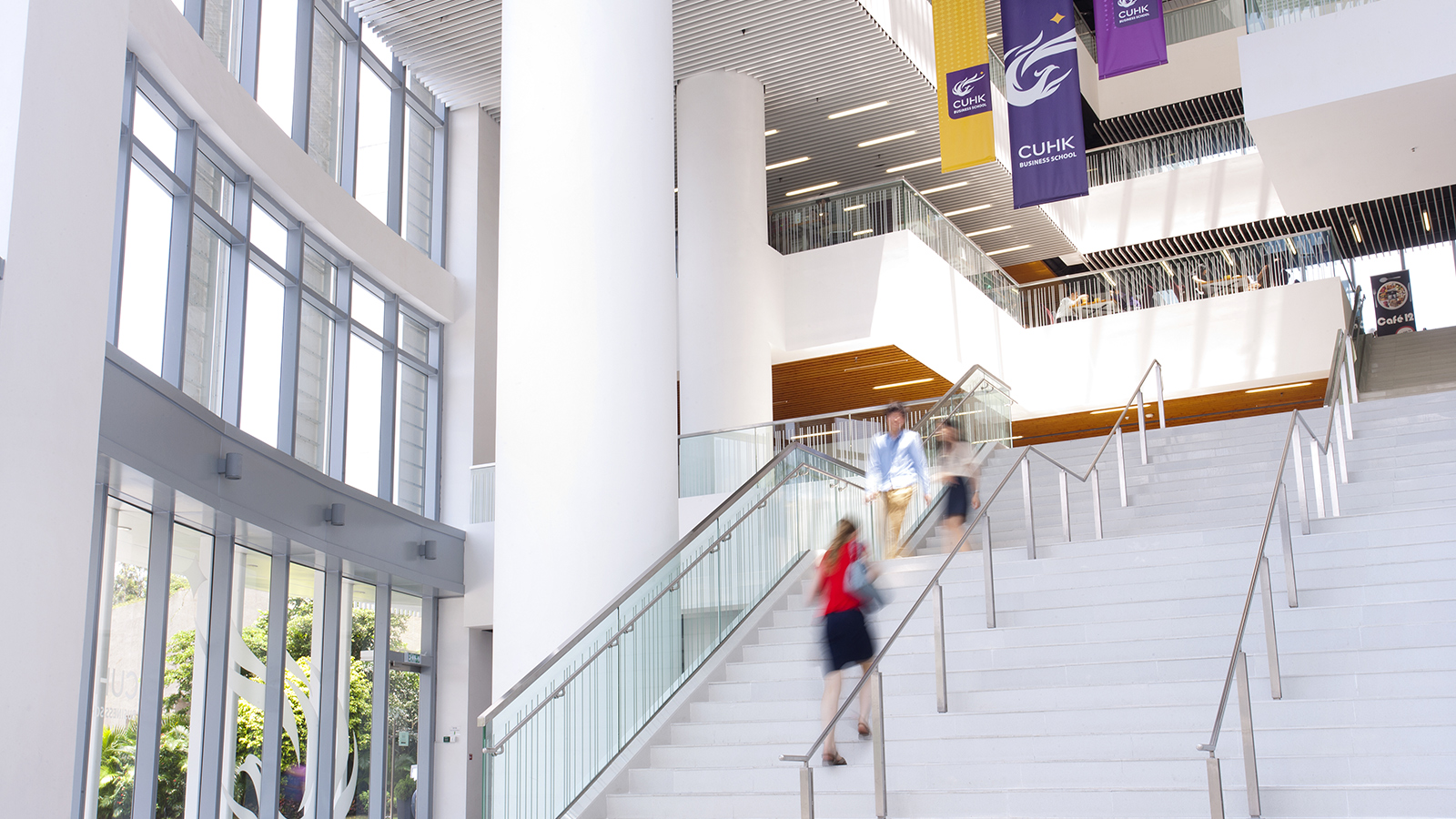
Research Interest
A common technical challenge encountered in many operations management models is that decision variables are truncated by some random variables and the decisions are made before the values of these random variables are realized, leading to non-convex minimization problems. To address this challenge, we develop a powerful transformation technique which converts a non-convex minimization problem to an equivalent convex one. We show that such a transformation enables us to prove the preservation of some desired structural properties, such as convexity, submodularity, and L-natural-convexity, under optimization operations, which are critical for identifying the structures of optimal policies, conducting comparative statics, and developing efficient algorithms. We then demonstrate the applications of our approach to several important models in inventory control and revenue management, with two concrete examples: joint inventory and transshipment control under random capacities, and capacity allocation in network revenue management. Additionally, we propose an efficient algorithm combining our transformation technique and piecewise-linear decision rule approach.