Three Essays on Operations Management
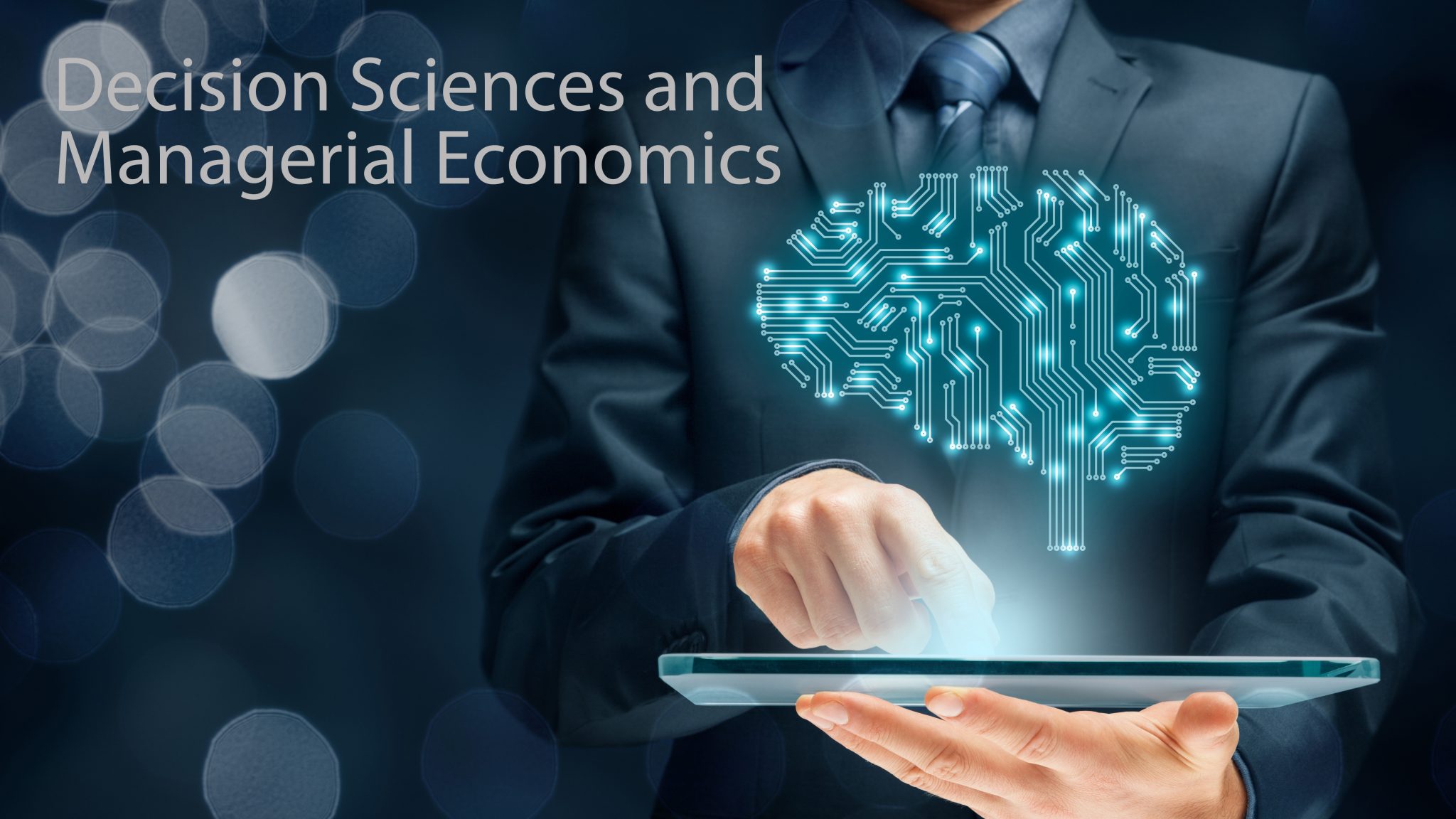
Abstract
Recent practice in operations management has demonstrated the importance of sourcing audits, product returns, and learning techniques. In this thesis, we present three essays that model and analyze such issues.
In the first essay, we discuss return policies in the framework of holiday seasons, which is motivated by the practice of extending return deadlines during holiday seasons by some retailers, such as Amazon, Apple, and BestBuy. Specifically, we study two strategies commonly adopted by retailers in the presence of strategic consumers who take into account return possibilities. The first strategy is that a retailer commits to its future prices, while the second is that the retailer employs dynamic pricing. We develop an analytical model and derive the optimal policies that are contingent on characteristics of retailers. We find that it is always optimal to allow return in regular season when the firm commits to its future prices. However, the optimal return policy is different when the firm employs dynamic pricing.
In the second essay, we investigate the supply chain responsibility and discuss strategies that can prevent the probable supplier-auditor collusion. In particular, we consider a supply chain in which a buyer sources from a supplier, while an auditor takes the task of auditing the quality of the supplier. We assume that a side contract is possible such that the auditor and the supplier may engage in a collusion if doing so is economically attractive. We develop a game-theoretic framework to model the collusion and examine two approaches to hindering the collusion. One approach is to readuit the auditing result, while the other is to adopt dual sourcing. We derive the equilibrium decisions of supply chain members with either approach in place and compare the effectiveness of the two approaches. Our analysis reveals that both approaches are able to hinder the collusion and reduce the risk of loss of orders due to violation. We also provide the optimal sourcing contract when the buyer faces a more practical but complex situation.
In the third essay, we leverage both statistical methods and machine learning techniques to predict outcomes of sport games with a focus on basketball. We collect data from NBA.com and some other sources for a time horizon of five years. We employ several commonly used methods, including logistic, linear discriminate analysis, quadratic discriminate analysis, Gaussian naïve Bayes, random forest, gradient boosting machines, and deep learning, to predict the outcomes of matches. We examine the accuracy of the predictions and make a comparison across the methods.