Understanding Customer Risk Adjustment in Commercial Banks: A Machine Learning-enabled Empirical Investigation
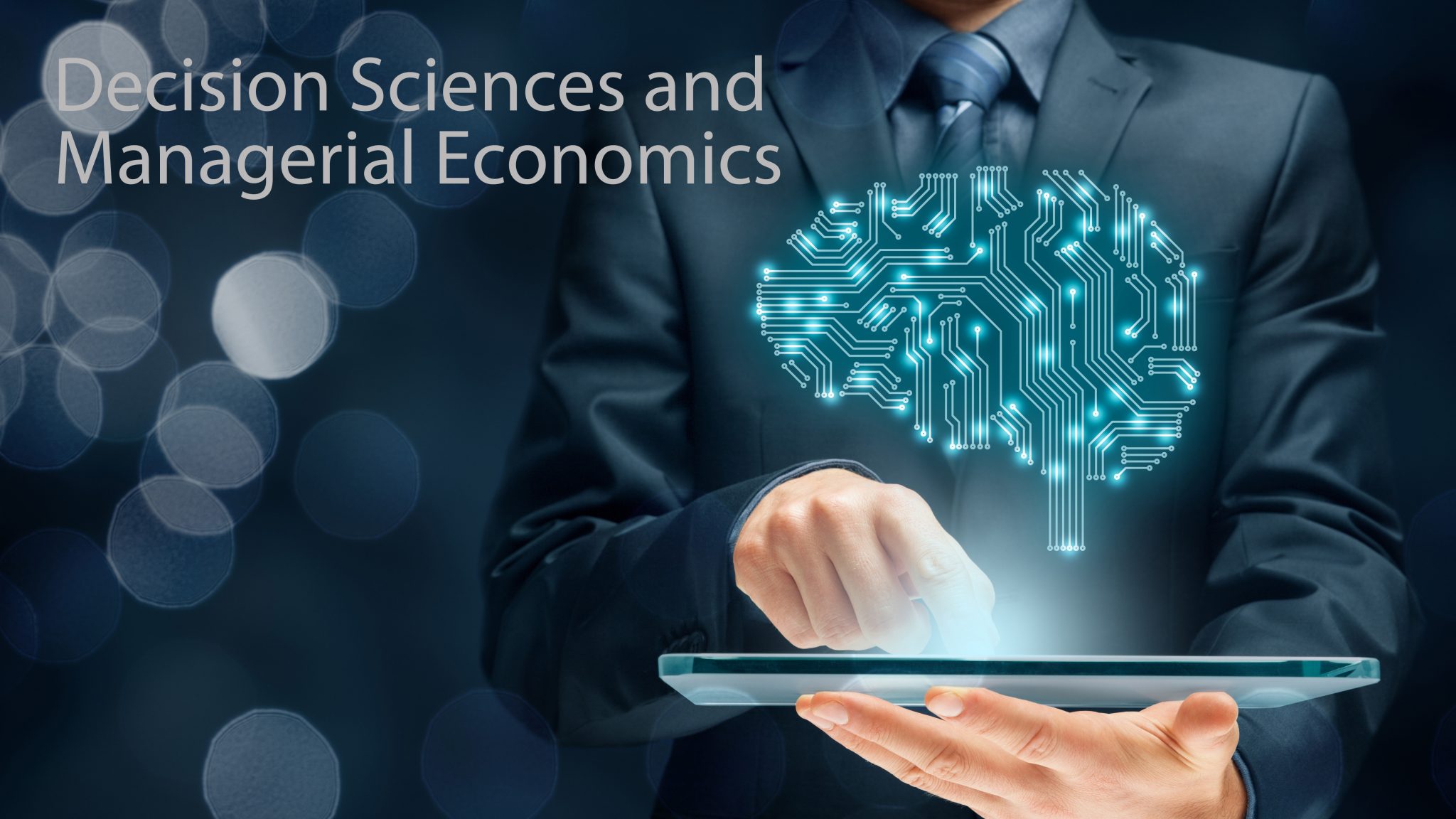
Understanding customers’ risk preferences is important for financial firms to effectively target offerings and manage relationships; however, assessing customers’ risk preference, particularly at scale and over time, is challenging. Lacking for efficient measures, we have limited understanding on risk preferences and the associated decision-making. Especially, little research has been carried out on when and how customers adjust their portfolio from the perspective of managing risk. To fill this research gap, we employ machine learning techniques to leverage rich data on customers’ behaviour that is commonly available to commercial banks to measure customers’ risk preferences. Using real-world data from a large commercial bank, we first identify customers’ risk preferences over time. Then, with the developed measure, we provide theoretical insights on how customers’ risk preferences and information consumption (about financial products) jointly affect their portfolio adjustment. Further, a field experiment in a marketing campaign shows that, telemarketers doubled their conversion rates based on our approach. These results suggest that the risk preference measure generated by machine learning has significant theoretical and practical implications.