Understanding Online User Opinions Using Economics and Technical Approaches
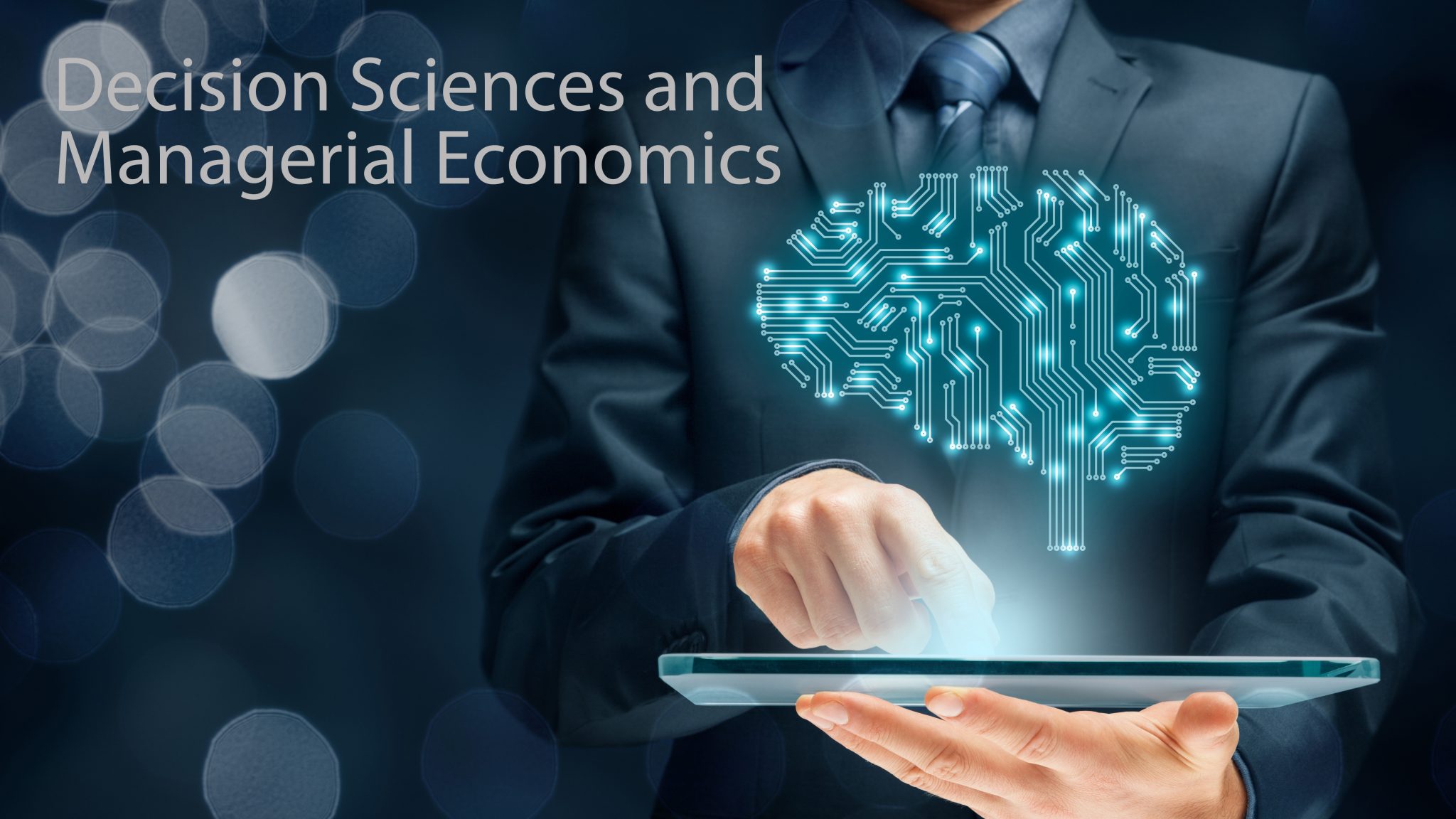
Abstract
In online platforms, users are frequently sharing digitalized opinions such as online reviews to express their thoughts and aid the purchase decision of others. With the widespread use of online platforms, information shared online now has a significant influence on potential customers’ decision-making processes as well as businesses’ revenues. Existing literature has examined various aspects of online platforms. However, there are some research gaps in current literature about online user opinions. For one thing, the online user opinions conveyed by ratings and texts would be unconsciously influenced in systematic ways. Therefore, the ratings and review contents may not exactly convey users’ objective opinions. For another, existing techniques that extract emotions from textual data are coarse-grained which are not comprehensive enough to be utilized in further empirical analysis. In this thesis, I am trying to fill these gaps using economics and technical approaches.
In the first study, leveraging econometric methods combining with machine learning techniques, I examine the causal impact of previous average rating on the rating and provision of emotional text in a subsequent online review, especially when the displayed rating is off from its true rating. Using online review data collected from Yelp, I apply natural language processing to measure emotions in each review and then use a regression discontinuity design by taking advantage of Yelp’s rounded rating display to study the causal effect. The primary results suggest that, a display of rounded-up rating (compared with that of rounded-down rating) has a negative impact on a subsequent rating and provision of pleasant emotional contents in a subsequent online review, while having a positive impact on the provision of unpleasant emotional contents in a subsequent review. Furthermore, I examine the moderating effect of prior reviews’ rating dispersion and information diversity on the main effect. The empirical results suggest that either lower rating dispersion or higher information diversity would intensify the main effect.
In the second study, I propose a hybrid deep learning model inspired by the human’s cognitive process in reading texts to help machines better understand online texts in a more human-like and interpretable way. This model incorporates three layers of attention modules, including cognitive word attention, sequential word attention, and sentence attention. These attention modules can provide insights into the model’s reasoning behind its prediction by automatically identifying information (e.g., word tokens) that is important for a specific task. Using a unique dataset which labels the level of eight discrete emotions for each online review from Yelp, the proposed deep learning model outperforms other widely adopted deep learning and traditional machine learning models in measuring the level of emotion provision for discrete emotions in textual data. Meanwhile, to ensure the robustness of the proposed model by performing text classification tasks on publicly available datasets, the proposed model has also shown its effectiveness for its unique ability to look into the black boxes of deep learning models as well as having good classification performance.