Bayesian Design Principles for Frequentist Sequential Learning
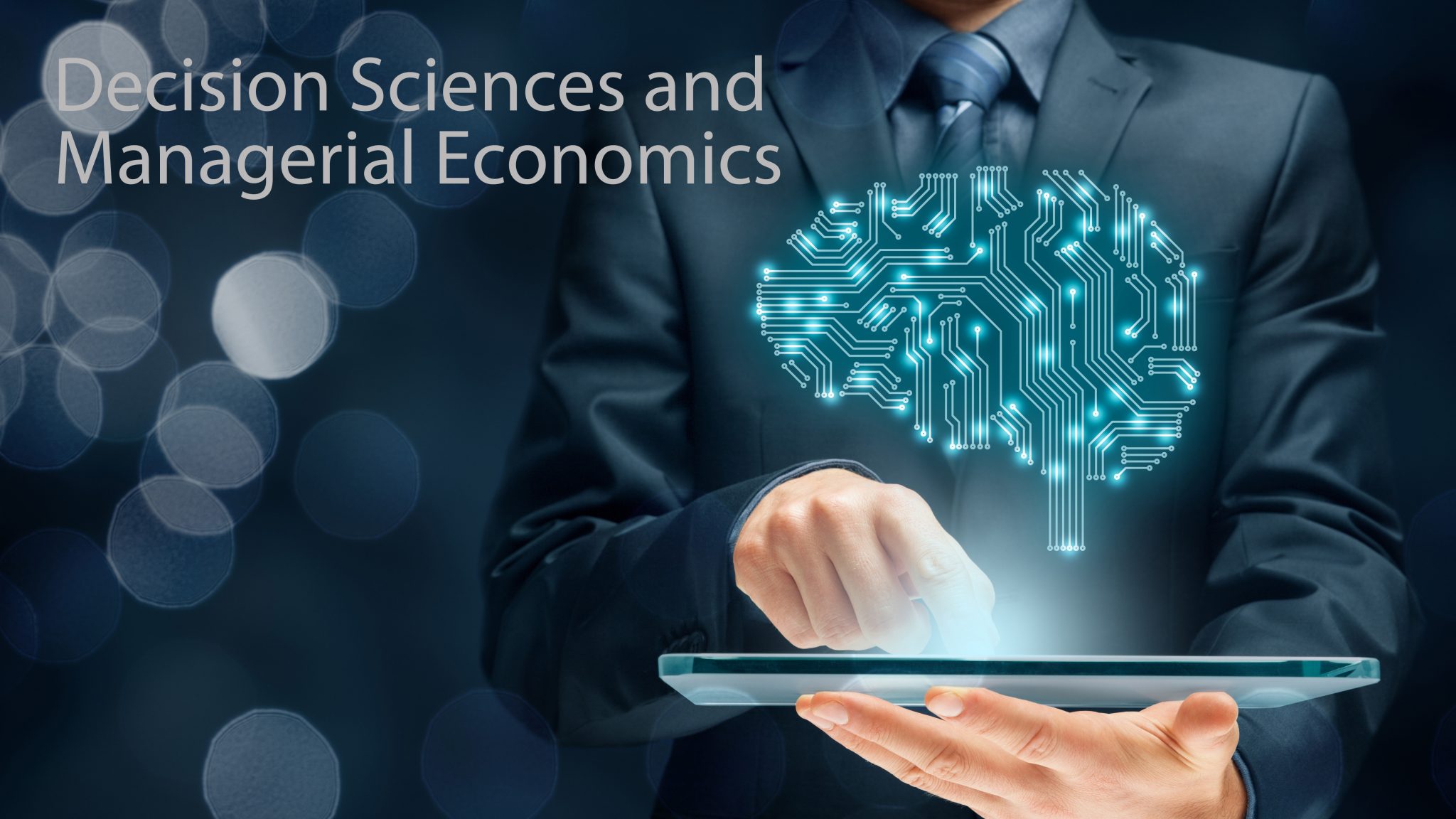
We propose a general framework to study frequentist sequential learning, where concepts from Bayesian inference are essential for algorithm design and regret analysis. We develop general algorithm design principles and study related complexity measures that apply in various bandit and reinforcement learning problems. In particular, we propose to design adaptive “algorithmic beliefs” instead of frequentist estimators; and make use of posterior updates instead of traditional frequentist decision rules. These posterior-based algorithms automatically adapt to non-stochastic environments, and their regret behaviour can often be shown to be best possible. Moreover, the algorithms are simple and often efficient to implement. In particular, we derive a novel algorithm for multi-armed bandit that achieves the “best-of-all-worlds” empirical performance in the stochastic, adversarial, and non-stationary environments. We also provide some unification and improved guarantees in infinite-armed bandit and reinforcement learning.